How to add extra word features other then word Embedding in Recurrent Neural Network model
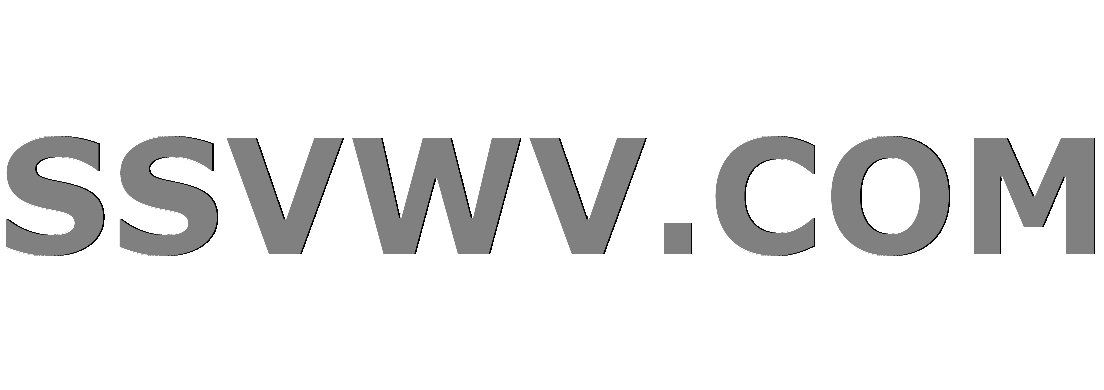
Multi tool use
$begingroup$
I am building a deep learning model for NLP. I am pretty comfortable with adding word embedding from word2vec or Glove vectors as extra word features but I wanted to add other word features like POS tag of a word, NER tag of word along with embedding as features. How can I do this. Should I give these word features by concatenating their vector with the word vectors. Or is there some other method. Please suggest.
deep-learning nlp rnn
$endgroup$
bumped to the homepage by Community♦ 2 mins ago
This question has answers that may be good or bad; the system has marked it active so that they can be reviewed.
add a comment |
$begingroup$
I am building a deep learning model for NLP. I am pretty comfortable with adding word embedding from word2vec or Glove vectors as extra word features but I wanted to add other word features like POS tag of a word, NER tag of word along with embedding as features. How can I do this. Should I give these word features by concatenating their vector with the word vectors. Or is there some other method. Please suggest.
deep-learning nlp rnn
$endgroup$
bumped to the homepage by Community♦ 2 mins ago
This question has answers that may be good or bad; the system has marked it active so that they can be reviewed.
1
$begingroup$
Yes, concatenate them.
$endgroup$
– Emre
Apr 28 '17 at 21:52
add a comment |
$begingroup$
I am building a deep learning model for NLP. I am pretty comfortable with adding word embedding from word2vec or Glove vectors as extra word features but I wanted to add other word features like POS tag of a word, NER tag of word along with embedding as features. How can I do this. Should I give these word features by concatenating their vector with the word vectors. Or is there some other method. Please suggest.
deep-learning nlp rnn
$endgroup$
I am building a deep learning model for NLP. I am pretty comfortable with adding word embedding from word2vec or Glove vectors as extra word features but I wanted to add other word features like POS tag of a word, NER tag of word along with embedding as features. How can I do this. Should I give these word features by concatenating their vector with the word vectors. Or is there some other method. Please suggest.
deep-learning nlp rnn
deep-learning nlp rnn
asked Apr 28 '17 at 14:51
PrayalankarPrayalankar
877
877
bumped to the homepage by Community♦ 2 mins ago
This question has answers that may be good or bad; the system has marked it active so that they can be reviewed.
bumped to the homepage by Community♦ 2 mins ago
This question has answers that may be good or bad; the system has marked it active so that they can be reviewed.
1
$begingroup$
Yes, concatenate them.
$endgroup$
– Emre
Apr 28 '17 at 21:52
add a comment |
1
$begingroup$
Yes, concatenate them.
$endgroup$
– Emre
Apr 28 '17 at 21:52
1
1
$begingroup$
Yes, concatenate them.
$endgroup$
– Emre
Apr 28 '17 at 21:52
$begingroup$
Yes, concatenate them.
$endgroup$
– Emre
Apr 28 '17 at 21:52
add a comment |
2 Answers
2
active
oldest
votes
$begingroup$
One option is to concatenate them, the second is to treat them as separate inputs. For example Keras offers such neural model: https://keras.io/getting-started/functional-api-guide/#multi-input-and-multi-output-models
$endgroup$
add a comment |
$begingroup$
I would concatenate them into a single input vector.
Essentially, your model treats each latent variable from the word embedding as a single feature (think about a regular ML model). Adding a couple to the end of this wouldn't hurt your performance too much.
Another option is to follow what @djstrong said, about multi-inputs. But I would start with just concatenating the extra variables at the end of your input vector.
$endgroup$
add a comment |
Your Answer
StackExchange.ifUsing("editor", function () {
return StackExchange.using("mathjaxEditing", function () {
StackExchange.MarkdownEditor.creationCallbacks.add(function (editor, postfix) {
StackExchange.mathjaxEditing.prepareWmdForMathJax(editor, postfix, [["$", "$"], ["\\(","\\)"]]);
});
});
}, "mathjax-editing");
StackExchange.ready(function() {
var channelOptions = {
tags: "".split(" "),
id: "557"
};
initTagRenderer("".split(" "), "".split(" "), channelOptions);
StackExchange.using("externalEditor", function() {
// Have to fire editor after snippets, if snippets enabled
if (StackExchange.settings.snippets.snippetsEnabled) {
StackExchange.using("snippets", function() {
createEditor();
});
}
else {
createEditor();
}
});
function createEditor() {
StackExchange.prepareEditor({
heartbeatType: 'answer',
autoActivateHeartbeat: false,
convertImagesToLinks: false,
noModals: true,
showLowRepImageUploadWarning: true,
reputationToPostImages: null,
bindNavPrevention: true,
postfix: "",
imageUploader: {
brandingHtml: "Powered by u003ca class="icon-imgur-white" href="https://imgur.com/"u003eu003c/au003e",
contentPolicyHtml: "User contributions licensed under u003ca href="https://creativecommons.org/licenses/by-sa/3.0/"u003ecc by-sa 3.0 with attribution requiredu003c/au003e u003ca href="https://stackoverflow.com/legal/content-policy"u003e(content policy)u003c/au003e",
allowUrls: true
},
onDemand: true,
discardSelector: ".discard-answer"
,immediatelyShowMarkdownHelp:true
});
}
});
Sign up or log in
StackExchange.ready(function () {
StackExchange.helpers.onClickDraftSave('#login-link');
});
Sign up using Google
Sign up using Facebook
Sign up using Email and Password
Post as a guest
Required, but never shown
StackExchange.ready(
function () {
StackExchange.openid.initPostLogin('.new-post-login', 'https%3a%2f%2fdatascience.stackexchange.com%2fquestions%2f18664%2fhow-to-add-extra-word-features-other-then-word-embedding-in-recurrent-neural-net%23new-answer', 'question_page');
}
);
Post as a guest
Required, but never shown
2 Answers
2
active
oldest
votes
2 Answers
2
active
oldest
votes
active
oldest
votes
active
oldest
votes
$begingroup$
One option is to concatenate them, the second is to treat them as separate inputs. For example Keras offers such neural model: https://keras.io/getting-started/functional-api-guide/#multi-input-and-multi-output-models
$endgroup$
add a comment |
$begingroup$
One option is to concatenate them, the second is to treat them as separate inputs. For example Keras offers such neural model: https://keras.io/getting-started/functional-api-guide/#multi-input-and-multi-output-models
$endgroup$
add a comment |
$begingroup$
One option is to concatenate them, the second is to treat them as separate inputs. For example Keras offers such neural model: https://keras.io/getting-started/functional-api-guide/#multi-input-and-multi-output-models
$endgroup$
One option is to concatenate them, the second is to treat them as separate inputs. For example Keras offers such neural model: https://keras.io/getting-started/functional-api-guide/#multi-input-and-multi-output-models
answered Jun 22 '18 at 10:42
djstrongdjstrong
212
212
add a comment |
add a comment |
$begingroup$
I would concatenate them into a single input vector.
Essentially, your model treats each latent variable from the word embedding as a single feature (think about a regular ML model). Adding a couple to the end of this wouldn't hurt your performance too much.
Another option is to follow what @djstrong said, about multi-inputs. But I would start with just concatenating the extra variables at the end of your input vector.
$endgroup$
add a comment |
$begingroup$
I would concatenate them into a single input vector.
Essentially, your model treats each latent variable from the word embedding as a single feature (think about a regular ML model). Adding a couple to the end of this wouldn't hurt your performance too much.
Another option is to follow what @djstrong said, about multi-inputs. But I would start with just concatenating the extra variables at the end of your input vector.
$endgroup$
add a comment |
$begingroup$
I would concatenate them into a single input vector.
Essentially, your model treats each latent variable from the word embedding as a single feature (think about a regular ML model). Adding a couple to the end of this wouldn't hurt your performance too much.
Another option is to follow what @djstrong said, about multi-inputs. But I would start with just concatenating the extra variables at the end of your input vector.
$endgroup$
I would concatenate them into a single input vector.
Essentially, your model treats each latent variable from the word embedding as a single feature (think about a regular ML model). Adding a couple to the end of this wouldn't hurt your performance too much.
Another option is to follow what @djstrong said, about multi-inputs. But I would start with just concatenating the extra variables at the end of your input vector.
answered Dec 20 '18 at 15:16


Arthur CamaraArthur Camara
101
101
add a comment |
add a comment |
Thanks for contributing an answer to Data Science Stack Exchange!
- Please be sure to answer the question. Provide details and share your research!
But avoid …
- Asking for help, clarification, or responding to other answers.
- Making statements based on opinion; back them up with references or personal experience.
Use MathJax to format equations. MathJax reference.
To learn more, see our tips on writing great answers.
Sign up or log in
StackExchange.ready(function () {
StackExchange.helpers.onClickDraftSave('#login-link');
});
Sign up using Google
Sign up using Facebook
Sign up using Email and Password
Post as a guest
Required, but never shown
StackExchange.ready(
function () {
StackExchange.openid.initPostLogin('.new-post-login', 'https%3a%2f%2fdatascience.stackexchange.com%2fquestions%2f18664%2fhow-to-add-extra-word-features-other-then-word-embedding-in-recurrent-neural-net%23new-answer', 'question_page');
}
);
Post as a guest
Required, but never shown
Sign up or log in
StackExchange.ready(function () {
StackExchange.helpers.onClickDraftSave('#login-link');
});
Sign up using Google
Sign up using Facebook
Sign up using Email and Password
Post as a guest
Required, but never shown
Sign up or log in
StackExchange.ready(function () {
StackExchange.helpers.onClickDraftSave('#login-link');
});
Sign up using Google
Sign up using Facebook
Sign up using Email and Password
Post as a guest
Required, but never shown
Sign up or log in
StackExchange.ready(function () {
StackExchange.helpers.onClickDraftSave('#login-link');
});
Sign up using Google
Sign up using Facebook
Sign up using Email and Password
Sign up using Google
Sign up using Facebook
Sign up using Email and Password
Post as a guest
Required, but never shown
Required, but never shown
Required, but never shown
Required, but never shown
Required, but never shown
Required, but never shown
Required, but never shown
Required, but never shown
Required, but never shown
iDE,p1,zzkb jzY,1hBWmLiTgTfS239XuLGTuP i9GMKx7aauJgHhavuM B7,vbHaRi,Ne8ucLGLWFtiBTTDCP,tHG0YVlZ37r
1
$begingroup$
Yes, concatenate them.
$endgroup$
– Emre
Apr 28 '17 at 21:52