Having trouble figuring out how loss was calculated for SQuAD task in BERT paper
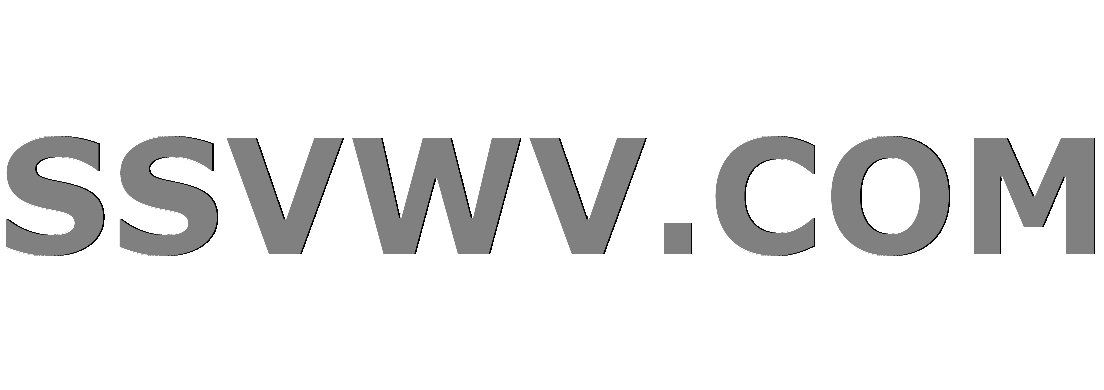
Multi tool use
$begingroup$
The BERT Paper
https://arxiv.org/pdf/1810.04805.pdf
Section 4.2 covers the SQuAD training.
So from my understanding, there are two extra parameters trained, they are two vectors with the same dimension as the hidden size, so the same dimensions as the contextualized embeddings in BERT. They are S (for start) and E (for End).
For each, a softmax is taken with S and each of the final contextualized embeddings to get a score for the correct Start position. And the same thing is done for E and the correct end position.
I get up to this part. But I am having trouble figuring out how the did the labeling and final loss calculations, which is described in this paragraph
"and the maximum scoring span is used as the prediction. The training objective is the loglikelihood of the correct start and end positions."
What do they mean by "maximum scoring span is used as the prediction"?
Furthermore, how does that play into "The training objective is the loglikelihood of the correct start and end positions"?
From this Source:
https://ljvmiranda921.github.io/notebook/2017/08/13/softmax-and-the-negative-log-likelihood/
It says the log-likelihood is only applied to the correct classes. So the we are only calculating the softmax for the correct positions only, Not any of the in correct positions.
If this interpretation is correct, then the loss will be
Loss = -Log( Softmax(S*T(predictedStart) / Sum(S*Ti) ) -Log( Softmax(E*T(predictedEnd) / Sum(S*Ti) )
machine-learning nlp loss-function
$endgroup$
add a comment |
$begingroup$
The BERT Paper
https://arxiv.org/pdf/1810.04805.pdf
Section 4.2 covers the SQuAD training.
So from my understanding, there are two extra parameters trained, they are two vectors with the same dimension as the hidden size, so the same dimensions as the contextualized embeddings in BERT. They are S (for start) and E (for End).
For each, a softmax is taken with S and each of the final contextualized embeddings to get a score for the correct Start position. And the same thing is done for E and the correct end position.
I get up to this part. But I am having trouble figuring out how the did the labeling and final loss calculations, which is described in this paragraph
"and the maximum scoring span is used as the prediction. The training objective is the loglikelihood of the correct start and end positions."
What do they mean by "maximum scoring span is used as the prediction"?
Furthermore, how does that play into "The training objective is the loglikelihood of the correct start and end positions"?
From this Source:
https://ljvmiranda921.github.io/notebook/2017/08/13/softmax-and-the-negative-log-likelihood/
It says the log-likelihood is only applied to the correct classes. So the we are only calculating the softmax for the correct positions only, Not any of the in correct positions.
If this interpretation is correct, then the loss will be
Loss = -Log( Softmax(S*T(predictedStart) / Sum(S*Ti) ) -Log( Softmax(E*T(predictedEnd) / Sum(S*Ti) )
machine-learning nlp loss-function
$endgroup$
add a comment |
$begingroup$
The BERT Paper
https://arxiv.org/pdf/1810.04805.pdf
Section 4.2 covers the SQuAD training.
So from my understanding, there are two extra parameters trained, they are two vectors with the same dimension as the hidden size, so the same dimensions as the contextualized embeddings in BERT. They are S (for start) and E (for End).
For each, a softmax is taken with S and each of the final contextualized embeddings to get a score for the correct Start position. And the same thing is done for E and the correct end position.
I get up to this part. But I am having trouble figuring out how the did the labeling and final loss calculations, which is described in this paragraph
"and the maximum scoring span is used as the prediction. The training objective is the loglikelihood of the correct start and end positions."
What do they mean by "maximum scoring span is used as the prediction"?
Furthermore, how does that play into "The training objective is the loglikelihood of the correct start and end positions"?
From this Source:
https://ljvmiranda921.github.io/notebook/2017/08/13/softmax-and-the-negative-log-likelihood/
It says the log-likelihood is only applied to the correct classes. So the we are only calculating the softmax for the correct positions only, Not any of the in correct positions.
If this interpretation is correct, then the loss will be
Loss = -Log( Softmax(S*T(predictedStart) / Sum(S*Ti) ) -Log( Softmax(E*T(predictedEnd) / Sum(S*Ti) )
machine-learning nlp loss-function
$endgroup$
The BERT Paper
https://arxiv.org/pdf/1810.04805.pdf
Section 4.2 covers the SQuAD training.
So from my understanding, there are two extra parameters trained, they are two vectors with the same dimension as the hidden size, so the same dimensions as the contextualized embeddings in BERT. They are S (for start) and E (for End).
For each, a softmax is taken with S and each of the final contextualized embeddings to get a score for the correct Start position. And the same thing is done for E and the correct end position.
I get up to this part. But I am having trouble figuring out how the did the labeling and final loss calculations, which is described in this paragraph
"and the maximum scoring span is used as the prediction. The training objective is the loglikelihood of the correct start and end positions."
What do they mean by "maximum scoring span is used as the prediction"?
Furthermore, how does that play into "The training objective is the loglikelihood of the correct start and end positions"?
From this Source:
https://ljvmiranda921.github.io/notebook/2017/08/13/softmax-and-the-negative-log-likelihood/
It says the log-likelihood is only applied to the correct classes. So the we are only calculating the softmax for the correct positions only, Not any of the in correct positions.
If this interpretation is correct, then the loss will be
Loss = -Log( Softmax(S*T(predictedStart) / Sum(S*Ti) ) -Log( Softmax(E*T(predictedEnd) / Sum(S*Ti) )
machine-learning nlp loss-function
machine-learning nlp loss-function
asked 11 mins ago
SantoshGupta7SantoshGupta7
1134
1134
add a comment |
add a comment |
0
active
oldest
votes
Your Answer
StackExchange.ready(function() {
var channelOptions = {
tags: "".split(" "),
id: "557"
};
initTagRenderer("".split(" "), "".split(" "), channelOptions);
StackExchange.using("externalEditor", function() {
// Have to fire editor after snippets, if snippets enabled
if (StackExchange.settings.snippets.snippetsEnabled) {
StackExchange.using("snippets", function() {
createEditor();
});
}
else {
createEditor();
}
});
function createEditor() {
StackExchange.prepareEditor({
heartbeatType: 'answer',
autoActivateHeartbeat: false,
convertImagesToLinks: false,
noModals: true,
showLowRepImageUploadWarning: true,
reputationToPostImages: null,
bindNavPrevention: true,
postfix: "",
imageUploader: {
brandingHtml: "Powered by u003ca class="icon-imgur-white" href="https://imgur.com/"u003eu003c/au003e",
contentPolicyHtml: "User contributions licensed under u003ca href="https://creativecommons.org/licenses/by-sa/3.0/"u003ecc by-sa 3.0 with attribution requiredu003c/au003e u003ca href="https://stackoverflow.com/legal/content-policy"u003e(content policy)u003c/au003e",
allowUrls: true
},
onDemand: true,
discardSelector: ".discard-answer"
,immediatelyShowMarkdownHelp:true
});
}
});
Sign up or log in
StackExchange.ready(function () {
StackExchange.helpers.onClickDraftSave('#login-link');
});
Sign up using Google
Sign up using Facebook
Sign up using Email and Password
Post as a guest
Required, but never shown
StackExchange.ready(
function () {
StackExchange.openid.initPostLogin('.new-post-login', 'https%3a%2f%2fdatascience.stackexchange.com%2fquestions%2f49621%2fhaving-trouble-figuring-out-how-loss-was-calculated-for-squad-task-in-bert-paper%23new-answer', 'question_page');
}
);
Post as a guest
Required, but never shown
0
active
oldest
votes
0
active
oldest
votes
active
oldest
votes
active
oldest
votes
Thanks for contributing an answer to Data Science Stack Exchange!
- Please be sure to answer the question. Provide details and share your research!
But avoid …
- Asking for help, clarification, or responding to other answers.
- Making statements based on opinion; back them up with references or personal experience.
Use MathJax to format equations. MathJax reference.
To learn more, see our tips on writing great answers.
Sign up or log in
StackExchange.ready(function () {
StackExchange.helpers.onClickDraftSave('#login-link');
});
Sign up using Google
Sign up using Facebook
Sign up using Email and Password
Post as a guest
Required, but never shown
StackExchange.ready(
function () {
StackExchange.openid.initPostLogin('.new-post-login', 'https%3a%2f%2fdatascience.stackexchange.com%2fquestions%2f49621%2fhaving-trouble-figuring-out-how-loss-was-calculated-for-squad-task-in-bert-paper%23new-answer', 'question_page');
}
);
Post as a guest
Required, but never shown
Sign up or log in
StackExchange.ready(function () {
StackExchange.helpers.onClickDraftSave('#login-link');
});
Sign up using Google
Sign up using Facebook
Sign up using Email and Password
Post as a guest
Required, but never shown
Sign up or log in
StackExchange.ready(function () {
StackExchange.helpers.onClickDraftSave('#login-link');
});
Sign up using Google
Sign up using Facebook
Sign up using Email and Password
Post as a guest
Required, but never shown
Sign up or log in
StackExchange.ready(function () {
StackExchange.helpers.onClickDraftSave('#login-link');
});
Sign up using Google
Sign up using Facebook
Sign up using Email and Password
Sign up using Google
Sign up using Facebook
Sign up using Email and Password
Post as a guest
Required, but never shown
Required, but never shown
Required, but never shown
Required, but never shown
Required, but never shown
Required, but never shown
Required, but never shown
Required, but never shown
Required, but never shown
TF5MZVKr,i6 3e6ZEixzwJ