Confusion on Delta Rule and Error
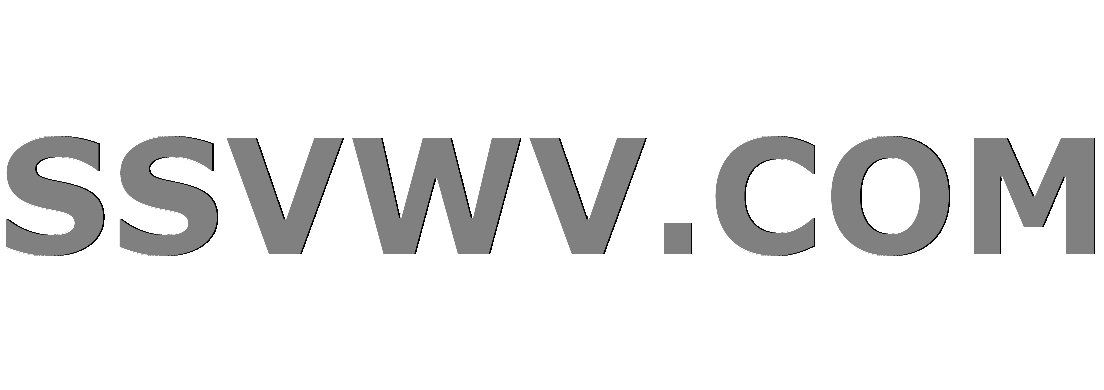
Multi tool use
$begingroup$
I'm currently reading Mitchell's book for Machine Learning, and he just started gradient descent. There's one part that's really confusing me.
At one point, he gives this equation for the error of a perceptron over a set of training examples.
$O_d$ is the actual output of $ vec{W} cdot vec{X}$, where $ vec{X}$ is the input vector and $vec{W}$ is the weights vector.
$t_d$ is the target output, what we want to get.
The sum over all the $D$ means we sum over every single $vec{X}$ we can input.
Okay, so far so good, I understand that.
However, he then gives this example:
But that is just not true!!!! That equation for the error does NOT give us a single minimum!!!
According to his previous rule, if we're considering the error for a single weight vector and a single training vector, the equation for the error would be:
$E(vec{w}) = frac{1}{2} * (t_d - (w_0 * x_0 + w_1 * x_1))^2$
Which has an infinite number of minimums!!! Every time $(w_0 * x_0 + w_1 * x_1) = t_d$
I graphed it here to show you:
In that picture, $x$ and $y$ are the two rows of the weight vector $vec{w}$.
Please help! I've been confused about this for the last three hours!
Thanks
machine-learning neural-network training gradient-descent perceptron
$endgroup$
add a comment |
$begingroup$
I'm currently reading Mitchell's book for Machine Learning, and he just started gradient descent. There's one part that's really confusing me.
At one point, he gives this equation for the error of a perceptron over a set of training examples.
$O_d$ is the actual output of $ vec{W} cdot vec{X}$, where $ vec{X}$ is the input vector and $vec{W}$ is the weights vector.
$t_d$ is the target output, what we want to get.
The sum over all the $D$ means we sum over every single $vec{X}$ we can input.
Okay, so far so good, I understand that.
However, he then gives this example:
But that is just not true!!!! That equation for the error does NOT give us a single minimum!!!
According to his previous rule, if we're considering the error for a single weight vector and a single training vector, the equation for the error would be:
$E(vec{w}) = frac{1}{2} * (t_d - (w_0 * x_0 + w_1 * x_1))^2$
Which has an infinite number of minimums!!! Every time $(w_0 * x_0 + w_1 * x_1) = t_d$
I graphed it here to show you:
In that picture, $x$ and $y$ are the two rows of the weight vector $vec{w}$.
Please help! I've been confused about this for the last three hours!
Thanks
machine-learning neural-network training gradient-descent perceptron
$endgroup$
add a comment |
$begingroup$
I'm currently reading Mitchell's book for Machine Learning, and he just started gradient descent. There's one part that's really confusing me.
At one point, he gives this equation for the error of a perceptron over a set of training examples.
$O_d$ is the actual output of $ vec{W} cdot vec{X}$, where $ vec{X}$ is the input vector and $vec{W}$ is the weights vector.
$t_d$ is the target output, what we want to get.
The sum over all the $D$ means we sum over every single $vec{X}$ we can input.
Okay, so far so good, I understand that.
However, he then gives this example:
But that is just not true!!!! That equation for the error does NOT give us a single minimum!!!
According to his previous rule, if we're considering the error for a single weight vector and a single training vector, the equation for the error would be:
$E(vec{w}) = frac{1}{2} * (t_d - (w_0 * x_0 + w_1 * x_1))^2$
Which has an infinite number of minimums!!! Every time $(w_0 * x_0 + w_1 * x_1) = t_d$
I graphed it here to show you:
In that picture, $x$ and $y$ are the two rows of the weight vector $vec{w}$.
Please help! I've been confused about this for the last three hours!
Thanks
machine-learning neural-network training gradient-descent perceptron
$endgroup$
I'm currently reading Mitchell's book for Machine Learning, and he just started gradient descent. There's one part that's really confusing me.
At one point, he gives this equation for the error of a perceptron over a set of training examples.
$O_d$ is the actual output of $ vec{W} cdot vec{X}$, where $ vec{X}$ is the input vector and $vec{W}$ is the weights vector.
$t_d$ is the target output, what we want to get.
The sum over all the $D$ means we sum over every single $vec{X}$ we can input.
Okay, so far so good, I understand that.
However, he then gives this example:
But that is just not true!!!! That equation for the error does NOT give us a single minimum!!!
According to his previous rule, if we're considering the error for a single weight vector and a single training vector, the equation for the error would be:
$E(vec{w}) = frac{1}{2} * (t_d - (w_0 * x_0 + w_1 * x_1))^2$
Which has an infinite number of minimums!!! Every time $(w_0 * x_0 + w_1 * x_1) = t_d$
I graphed it here to show you:
In that picture, $x$ and $y$ are the two rows of the weight vector $vec{w}$.
Please help! I've been confused about this for the last three hours!
Thanks
machine-learning neural-network training gradient-descent perceptron
machine-learning neural-network training gradient-descent perceptron
asked 4 mins ago
Joshua RonisJoshua Ronis
1334
1334
add a comment |
add a comment |
0
active
oldest
votes
Your Answer
StackExchange.ifUsing("editor", function () {
return StackExchange.using("mathjaxEditing", function () {
StackExchange.MarkdownEditor.creationCallbacks.add(function (editor, postfix) {
StackExchange.mathjaxEditing.prepareWmdForMathJax(editor, postfix, [["$", "$"], ["\\(","\\)"]]);
});
});
}, "mathjax-editing");
StackExchange.ready(function() {
var channelOptions = {
tags: "".split(" "),
id: "557"
};
initTagRenderer("".split(" "), "".split(" "), channelOptions);
StackExchange.using("externalEditor", function() {
// Have to fire editor after snippets, if snippets enabled
if (StackExchange.settings.snippets.snippetsEnabled) {
StackExchange.using("snippets", function() {
createEditor();
});
}
else {
createEditor();
}
});
function createEditor() {
StackExchange.prepareEditor({
heartbeatType: 'answer',
autoActivateHeartbeat: false,
convertImagesToLinks: false,
noModals: true,
showLowRepImageUploadWarning: true,
reputationToPostImages: null,
bindNavPrevention: true,
postfix: "",
imageUploader: {
brandingHtml: "Powered by u003ca class="icon-imgur-white" href="https://imgur.com/"u003eu003c/au003e",
contentPolicyHtml: "User contributions licensed under u003ca href="https://creativecommons.org/licenses/by-sa/3.0/"u003ecc by-sa 3.0 with attribution requiredu003c/au003e u003ca href="https://stackoverflow.com/legal/content-policy"u003e(content policy)u003c/au003e",
allowUrls: true
},
onDemand: true,
discardSelector: ".discard-answer"
,immediatelyShowMarkdownHelp:true
});
}
});
Sign up or log in
StackExchange.ready(function () {
StackExchange.helpers.onClickDraftSave('#login-link');
});
Sign up using Google
Sign up using Facebook
Sign up using Email and Password
Post as a guest
Required, but never shown
StackExchange.ready(
function () {
StackExchange.openid.initPostLogin('.new-post-login', 'https%3a%2f%2fdatascience.stackexchange.com%2fquestions%2f44402%2fconfusion-on-delta-rule-and-error%23new-answer', 'question_page');
}
);
Post as a guest
Required, but never shown
0
active
oldest
votes
0
active
oldest
votes
active
oldest
votes
active
oldest
votes
Thanks for contributing an answer to Data Science Stack Exchange!
- Please be sure to answer the question. Provide details and share your research!
But avoid …
- Asking for help, clarification, or responding to other answers.
- Making statements based on opinion; back them up with references or personal experience.
Use MathJax to format equations. MathJax reference.
To learn more, see our tips on writing great answers.
Sign up or log in
StackExchange.ready(function () {
StackExchange.helpers.onClickDraftSave('#login-link');
});
Sign up using Google
Sign up using Facebook
Sign up using Email and Password
Post as a guest
Required, but never shown
StackExchange.ready(
function () {
StackExchange.openid.initPostLogin('.new-post-login', 'https%3a%2f%2fdatascience.stackexchange.com%2fquestions%2f44402%2fconfusion-on-delta-rule-and-error%23new-answer', 'question_page');
}
);
Post as a guest
Required, but never shown
Sign up or log in
StackExchange.ready(function () {
StackExchange.helpers.onClickDraftSave('#login-link');
});
Sign up using Google
Sign up using Facebook
Sign up using Email and Password
Post as a guest
Required, but never shown
Sign up or log in
StackExchange.ready(function () {
StackExchange.helpers.onClickDraftSave('#login-link');
});
Sign up using Google
Sign up using Facebook
Sign up using Email and Password
Post as a guest
Required, but never shown
Sign up or log in
StackExchange.ready(function () {
StackExchange.helpers.onClickDraftSave('#login-link');
});
Sign up using Google
Sign up using Facebook
Sign up using Email and Password
Sign up using Google
Sign up using Facebook
Sign up using Email and Password
Post as a guest
Required, but never shown
Required, but never shown
Required, but never shown
Required, but never shown
Required, but never shown
Required, but never shown
Required, but never shown
Required, but never shown
Required, but never shown
UXDCt2,xu rYgIV 16TY tEOhQKBCttBRaYB,GK2cRrEmbcOaJ Ibj clVEBw,cPf9WH5BH3XIgx OlVkL5,6ks,IYhM3hYf5wr7Qe