How to find a transformation of a random process X so it has distribution of a reference process Y?
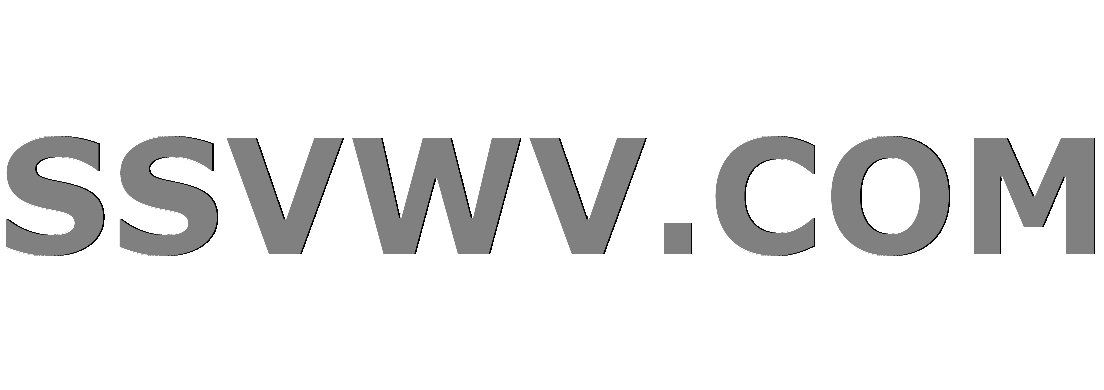
Multi tool use
$begingroup$
I was thinking to use GAN or KL Divergence as a loss function to do the following:
Let $X sim D$ where $D$ is some distribution. Assume we know a reference asymptotic distribution $Y sim D_2$.
We would like to find a polynomial transformation of $X to f(X)$ such that $f(X) sim Y sim D_2$.
For the case of $Y sim D_2=N(0,1)$ the network potentially will find the z-score normalization or some mapping $f$, so $f(x) sim Y$.
I would like to get advice how to formalize this problem in order to be able to solve it using Neural Network.
machine-learning deep-learning statistics
$endgroup$
|
show 1 more comment
$begingroup$
I was thinking to use GAN or KL Divergence as a loss function to do the following:
Let $X sim D$ where $D$ is some distribution. Assume we know a reference asymptotic distribution $Y sim D_2$.
We would like to find a polynomial transformation of $X to f(X)$ such that $f(X) sim Y sim D_2$.
For the case of $Y sim D_2=N(0,1)$ the network potentially will find the z-score normalization or some mapping $f$, so $f(x) sim Y$.
I would like to get advice how to formalize this problem in order to be able to solve it using Neural Network.
machine-learning deep-learning statistics
$endgroup$
$begingroup$
What is the "polynomial" constraint for? Finite sum of polynomials? Generally, Neural networks can be approximated with infinite sum of polynomials.
$endgroup$
– Esmailian
13 hours ago
$begingroup$
@Esmailian, I would like the "network" to find a point-wise transformation, $f$, such that ${f(x) | forall x in X}$ will have the same distribution as $Y$. Yes I think we can assume a maximal degree for the polynomial (e.g. N=10) What do you mean by:Generally, Neural networks can be approximated with infinite sum of polynomials.
$endgroup$
– 0x90
12 hours ago
1
$begingroup$
Take a look at these kind of transformations: QuantileTransformer.
$endgroup$
– Esmailian
12 hours ago
$begingroup$
@Esmailian this is great for the normal distribution case. However, I wanted to approach it by minimizing the loss function of the KL div between two sequences.
$endgroup$
– 0x90
12 hours ago
$begingroup$
I meant you cannot place an upper bound on the number of polynomials when you use Neural Networks with non-linear activation functions to represent $f$.
$endgroup$
– Esmailian
12 hours ago
|
show 1 more comment
$begingroup$
I was thinking to use GAN or KL Divergence as a loss function to do the following:
Let $X sim D$ where $D$ is some distribution. Assume we know a reference asymptotic distribution $Y sim D_2$.
We would like to find a polynomial transformation of $X to f(X)$ such that $f(X) sim Y sim D_2$.
For the case of $Y sim D_2=N(0,1)$ the network potentially will find the z-score normalization or some mapping $f$, so $f(x) sim Y$.
I would like to get advice how to formalize this problem in order to be able to solve it using Neural Network.
machine-learning deep-learning statistics
$endgroup$
I was thinking to use GAN or KL Divergence as a loss function to do the following:
Let $X sim D$ where $D$ is some distribution. Assume we know a reference asymptotic distribution $Y sim D_2$.
We would like to find a polynomial transformation of $X to f(X)$ such that $f(X) sim Y sim D_2$.
For the case of $Y sim D_2=N(0,1)$ the network potentially will find the z-score normalization or some mapping $f$, so $f(x) sim Y$.
I would like to get advice how to formalize this problem in order to be able to solve it using Neural Network.
machine-learning deep-learning statistics
machine-learning deep-learning statistics
edited 1 min ago
0x90
asked yesterday


0x900x90
1237
1237
$begingroup$
What is the "polynomial" constraint for? Finite sum of polynomials? Generally, Neural networks can be approximated with infinite sum of polynomials.
$endgroup$
– Esmailian
13 hours ago
$begingroup$
@Esmailian, I would like the "network" to find a point-wise transformation, $f$, such that ${f(x) | forall x in X}$ will have the same distribution as $Y$. Yes I think we can assume a maximal degree for the polynomial (e.g. N=10) What do you mean by:Generally, Neural networks can be approximated with infinite sum of polynomials.
$endgroup$
– 0x90
12 hours ago
1
$begingroup$
Take a look at these kind of transformations: QuantileTransformer.
$endgroup$
– Esmailian
12 hours ago
$begingroup$
@Esmailian this is great for the normal distribution case. However, I wanted to approach it by minimizing the loss function of the KL div between two sequences.
$endgroup$
– 0x90
12 hours ago
$begingroup$
I meant you cannot place an upper bound on the number of polynomials when you use Neural Networks with non-linear activation functions to represent $f$.
$endgroup$
– Esmailian
12 hours ago
|
show 1 more comment
$begingroup$
What is the "polynomial" constraint for? Finite sum of polynomials? Generally, Neural networks can be approximated with infinite sum of polynomials.
$endgroup$
– Esmailian
13 hours ago
$begingroup$
@Esmailian, I would like the "network" to find a point-wise transformation, $f$, such that ${f(x) | forall x in X}$ will have the same distribution as $Y$. Yes I think we can assume a maximal degree for the polynomial (e.g. N=10) What do you mean by:Generally, Neural networks can be approximated with infinite sum of polynomials.
$endgroup$
– 0x90
12 hours ago
1
$begingroup$
Take a look at these kind of transformations: QuantileTransformer.
$endgroup$
– Esmailian
12 hours ago
$begingroup$
@Esmailian this is great for the normal distribution case. However, I wanted to approach it by minimizing the loss function of the KL div between two sequences.
$endgroup$
– 0x90
12 hours ago
$begingroup$
I meant you cannot place an upper bound on the number of polynomials when you use Neural Networks with non-linear activation functions to represent $f$.
$endgroup$
– Esmailian
12 hours ago
$begingroup$
What is the "polynomial" constraint for? Finite sum of polynomials? Generally, Neural networks can be approximated with infinite sum of polynomials.
$endgroup$
– Esmailian
13 hours ago
$begingroup$
What is the "polynomial" constraint for? Finite sum of polynomials? Generally, Neural networks can be approximated with infinite sum of polynomials.
$endgroup$
– Esmailian
13 hours ago
$begingroup$
@Esmailian, I would like the "network" to find a point-wise transformation, $f$, such that ${f(x) | forall x in X}$ will have the same distribution as $Y$. Yes I think we can assume a maximal degree for the polynomial (e.g. N=10) What do you mean by:
Generally, Neural networks can be approximated with infinite sum of polynomials.
$endgroup$
– 0x90
12 hours ago
$begingroup$
@Esmailian, I would like the "network" to find a point-wise transformation, $f$, such that ${f(x) | forall x in X}$ will have the same distribution as $Y$. Yes I think we can assume a maximal degree for the polynomial (e.g. N=10) What do you mean by:
Generally, Neural networks can be approximated with infinite sum of polynomials.
$endgroup$
– 0x90
12 hours ago
1
1
$begingroup$
Take a look at these kind of transformations: QuantileTransformer.
$endgroup$
– Esmailian
12 hours ago
$begingroup$
Take a look at these kind of transformations: QuantileTransformer.
$endgroup$
– Esmailian
12 hours ago
$begingroup$
@Esmailian this is great for the normal distribution case. However, I wanted to approach it by minimizing the loss function of the KL div between two sequences.
$endgroup$
– 0x90
12 hours ago
$begingroup$
@Esmailian this is great for the normal distribution case. However, I wanted to approach it by minimizing the loss function of the KL div between two sequences.
$endgroup$
– 0x90
12 hours ago
$begingroup$
I meant you cannot place an upper bound on the number of polynomials when you use Neural Networks with non-linear activation functions to represent $f$.
$endgroup$
– Esmailian
12 hours ago
$begingroup$
I meant you cannot place an upper bound on the number of polynomials when you use Neural Networks with non-linear activation functions to represent $f$.
$endgroup$
– Esmailian
12 hours ago
|
show 1 more comment
0
active
oldest
votes
Your Answer
StackExchange.ifUsing("editor", function () {
return StackExchange.using("mathjaxEditing", function () {
StackExchange.MarkdownEditor.creationCallbacks.add(function (editor, postfix) {
StackExchange.mathjaxEditing.prepareWmdForMathJax(editor, postfix, [["$", "$"], ["\\(","\\)"]]);
});
});
}, "mathjax-editing");
StackExchange.ready(function() {
var channelOptions = {
tags: "".split(" "),
id: "557"
};
initTagRenderer("".split(" "), "".split(" "), channelOptions);
StackExchange.using("externalEditor", function() {
// Have to fire editor after snippets, if snippets enabled
if (StackExchange.settings.snippets.snippetsEnabled) {
StackExchange.using("snippets", function() {
createEditor();
});
}
else {
createEditor();
}
});
function createEditor() {
StackExchange.prepareEditor({
heartbeatType: 'answer',
autoActivateHeartbeat: false,
convertImagesToLinks: false,
noModals: true,
showLowRepImageUploadWarning: true,
reputationToPostImages: null,
bindNavPrevention: true,
postfix: "",
imageUploader: {
brandingHtml: "Powered by u003ca class="icon-imgur-white" href="https://imgur.com/"u003eu003c/au003e",
contentPolicyHtml: "User contributions licensed under u003ca href="https://creativecommons.org/licenses/by-sa/3.0/"u003ecc by-sa 3.0 with attribution requiredu003c/au003e u003ca href="https://stackoverflow.com/legal/content-policy"u003e(content policy)u003c/au003e",
allowUrls: true
},
onDemand: true,
discardSelector: ".discard-answer"
,immediatelyShowMarkdownHelp:true
});
}
});
Sign up or log in
StackExchange.ready(function () {
StackExchange.helpers.onClickDraftSave('#login-link');
});
Sign up using Google
Sign up using Facebook
Sign up using Email and Password
Post as a guest
Required, but never shown
StackExchange.ready(
function () {
StackExchange.openid.initPostLogin('.new-post-login', 'https%3a%2f%2fdatascience.stackexchange.com%2fquestions%2f48241%2fhow-to-find-a-transformation-of-a-random-process-x-so-it-has-distribution-of-a-r%23new-answer', 'question_page');
}
);
Post as a guest
Required, but never shown
0
active
oldest
votes
0
active
oldest
votes
active
oldest
votes
active
oldest
votes
Thanks for contributing an answer to Data Science Stack Exchange!
- Please be sure to answer the question. Provide details and share your research!
But avoid …
- Asking for help, clarification, or responding to other answers.
- Making statements based on opinion; back them up with references or personal experience.
Use MathJax to format equations. MathJax reference.
To learn more, see our tips on writing great answers.
Sign up or log in
StackExchange.ready(function () {
StackExchange.helpers.onClickDraftSave('#login-link');
});
Sign up using Google
Sign up using Facebook
Sign up using Email and Password
Post as a guest
Required, but never shown
StackExchange.ready(
function () {
StackExchange.openid.initPostLogin('.new-post-login', 'https%3a%2f%2fdatascience.stackexchange.com%2fquestions%2f48241%2fhow-to-find-a-transformation-of-a-random-process-x-so-it-has-distribution-of-a-r%23new-answer', 'question_page');
}
);
Post as a guest
Required, but never shown
Sign up or log in
StackExchange.ready(function () {
StackExchange.helpers.onClickDraftSave('#login-link');
});
Sign up using Google
Sign up using Facebook
Sign up using Email and Password
Post as a guest
Required, but never shown
Sign up or log in
StackExchange.ready(function () {
StackExchange.helpers.onClickDraftSave('#login-link');
});
Sign up using Google
Sign up using Facebook
Sign up using Email and Password
Post as a guest
Required, but never shown
Sign up or log in
StackExchange.ready(function () {
StackExchange.helpers.onClickDraftSave('#login-link');
});
Sign up using Google
Sign up using Facebook
Sign up using Email and Password
Sign up using Google
Sign up using Facebook
Sign up using Email and Password
Post as a guest
Required, but never shown
Required, but never shown
Required, but never shown
Required, but never shown
Required, but never shown
Required, but never shown
Required, but never shown
Required, but never shown
Required, but never shown
4gyBQhZnCG,tbC0X,hc,Cth,kz53IKJTmrGHqN8G vBGaEvRKM GHy8bJN8pNyewurw79atY,IZ2tSznuvI
$begingroup$
What is the "polynomial" constraint for? Finite sum of polynomials? Generally, Neural networks can be approximated with infinite sum of polynomials.
$endgroup$
– Esmailian
13 hours ago
$begingroup$
@Esmailian, I would like the "network" to find a point-wise transformation, $f$, such that ${f(x) | forall x in X}$ will have the same distribution as $Y$. Yes I think we can assume a maximal degree for the polynomial (e.g. N=10) What do you mean by:
Generally, Neural networks can be approximated with infinite sum of polynomials.
$endgroup$
– 0x90
12 hours ago
1
$begingroup$
Take a look at these kind of transformations: QuantileTransformer.
$endgroup$
– Esmailian
12 hours ago
$begingroup$
@Esmailian this is great for the normal distribution case. However, I wanted to approach it by minimizing the loss function of the KL div between two sequences.
$endgroup$
– 0x90
12 hours ago
$begingroup$
I meant you cannot place an upper bound on the number of polynomials when you use Neural Networks with non-linear activation functions to represent $f$.
$endgroup$
– Esmailian
12 hours ago