Vanishing gradient problem for recent stochastic recurrent neural networks
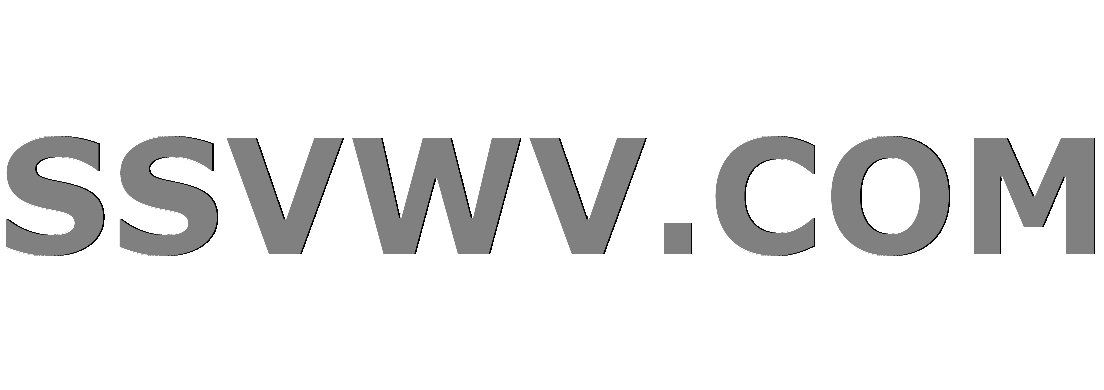
Multi tool use
$begingroup$
Recently, I've found some papers about generative recurrent models. All have attached sub-networks like prior/encoder/decoder/etc. to well-known LSTM cell for composing an aggregation of new-type RNN cell.
I am just curious about whether the gradient vanishing/exploding happens or not to those new RNN cell. Isn't there any problem about that kind of combination?
References:
It seems like they all have similar pattern as mentioned above.
A Recurrent Latent Variable Model for Sequential Data
Learning Stochastic Recurrent Networks
Z-Forcing: Training Stochastic Recurrent Networks
Pseudocode
The pseudocode for recurrent architecture is below:
def new_rnncell_call(x, htm1):
#prior_net/posterior_net/decoder_net is single layer or mlp each
q_prior = prior_net(htm1) # prior step
q = posterior_net([htm1, x]) # inference step
z = sample_from(q) # reparameterization trick
target_dist = decoder_net(z) # generation step
ht = innerLSTM([z, x], htm1) # recurrent step
return [q_prior, q, target_dist], ht
What concerns me are those naked weights outside of well-known LSTM (or GRU etc.) cell during processing bptt without any gating logic for activations as the weights inside LSTM. For me, this looks not similar to stacked-rnn layers or additional dense layers just to outputs.
Doesn't that have any gradient vanishing/exploding problem?
python deep-learning gradient-descent recurrent-neural-net
New contributor
Sehee Park is a new contributor to this site. Take care in asking for clarification, commenting, and answering.
Check out our Code of Conduct.
$endgroup$
add a comment |
$begingroup$
Recently, I've found some papers about generative recurrent models. All have attached sub-networks like prior/encoder/decoder/etc. to well-known LSTM cell for composing an aggregation of new-type RNN cell.
I am just curious about whether the gradient vanishing/exploding happens or not to those new RNN cell. Isn't there any problem about that kind of combination?
References:
It seems like they all have similar pattern as mentioned above.
A Recurrent Latent Variable Model for Sequential Data
Learning Stochastic Recurrent Networks
Z-Forcing: Training Stochastic Recurrent Networks
Pseudocode
The pseudocode for recurrent architecture is below:
def new_rnncell_call(x, htm1):
#prior_net/posterior_net/decoder_net is single layer or mlp each
q_prior = prior_net(htm1) # prior step
q = posterior_net([htm1, x]) # inference step
z = sample_from(q) # reparameterization trick
target_dist = decoder_net(z) # generation step
ht = innerLSTM([z, x], htm1) # recurrent step
return [q_prior, q, target_dist], ht
What concerns me are those naked weights outside of well-known LSTM (or GRU etc.) cell during processing bptt without any gating logic for activations as the weights inside LSTM. For me, this looks not similar to stacked-rnn layers or additional dense layers just to outputs.
Doesn't that have any gradient vanishing/exploding problem?
python deep-learning gradient-descent recurrent-neural-net
New contributor
Sehee Park is a new contributor to this site. Take care in asking for clarification, commenting, and answering.
Check out our Code of Conduct.
$endgroup$
add a comment |
$begingroup$
Recently, I've found some papers about generative recurrent models. All have attached sub-networks like prior/encoder/decoder/etc. to well-known LSTM cell for composing an aggregation of new-type RNN cell.
I am just curious about whether the gradient vanishing/exploding happens or not to those new RNN cell. Isn't there any problem about that kind of combination?
References:
It seems like they all have similar pattern as mentioned above.
A Recurrent Latent Variable Model for Sequential Data
Learning Stochastic Recurrent Networks
Z-Forcing: Training Stochastic Recurrent Networks
Pseudocode
The pseudocode for recurrent architecture is below:
def new_rnncell_call(x, htm1):
#prior_net/posterior_net/decoder_net is single layer or mlp each
q_prior = prior_net(htm1) # prior step
q = posterior_net([htm1, x]) # inference step
z = sample_from(q) # reparameterization trick
target_dist = decoder_net(z) # generation step
ht = innerLSTM([z, x], htm1) # recurrent step
return [q_prior, q, target_dist], ht
What concerns me are those naked weights outside of well-known LSTM (or GRU etc.) cell during processing bptt without any gating logic for activations as the weights inside LSTM. For me, this looks not similar to stacked-rnn layers or additional dense layers just to outputs.
Doesn't that have any gradient vanishing/exploding problem?
python deep-learning gradient-descent recurrent-neural-net
New contributor
Sehee Park is a new contributor to this site. Take care in asking for clarification, commenting, and answering.
Check out our Code of Conduct.
$endgroup$
Recently, I've found some papers about generative recurrent models. All have attached sub-networks like prior/encoder/decoder/etc. to well-known LSTM cell for composing an aggregation of new-type RNN cell.
I am just curious about whether the gradient vanishing/exploding happens or not to those new RNN cell. Isn't there any problem about that kind of combination?
References:
It seems like they all have similar pattern as mentioned above.
A Recurrent Latent Variable Model for Sequential Data
Learning Stochastic Recurrent Networks
Z-Forcing: Training Stochastic Recurrent Networks
Pseudocode
The pseudocode for recurrent architecture is below:
def new_rnncell_call(x, htm1):
#prior_net/posterior_net/decoder_net is single layer or mlp each
q_prior = prior_net(htm1) # prior step
q = posterior_net([htm1, x]) # inference step
z = sample_from(q) # reparameterization trick
target_dist = decoder_net(z) # generation step
ht = innerLSTM([z, x], htm1) # recurrent step
return [q_prior, q, target_dist], ht
What concerns me are those naked weights outside of well-known LSTM (or GRU etc.) cell during processing bptt without any gating logic for activations as the weights inside LSTM. For me, this looks not similar to stacked-rnn layers or additional dense layers just to outputs.
Doesn't that have any gradient vanishing/exploding problem?
python deep-learning gradient-descent recurrent-neural-net
python deep-learning gradient-descent recurrent-neural-net
New contributor
Sehee Park is a new contributor to this site. Take care in asking for clarification, commenting, and answering.
Check out our Code of Conduct.
New contributor
Sehee Park is a new contributor to this site. Take care in asking for clarification, commenting, and answering.
Check out our Code of Conduct.
New contributor
Sehee Park is a new contributor to this site. Take care in asking for clarification, commenting, and answering.
Check out our Code of Conduct.
asked 5 mins ago
Sehee ParkSehee Park
1
1
New contributor
Sehee Park is a new contributor to this site. Take care in asking for clarification, commenting, and answering.
Check out our Code of Conduct.
New contributor
Sehee Park is a new contributor to this site. Take care in asking for clarification, commenting, and answering.
Check out our Code of Conduct.
Sehee Park is a new contributor to this site. Take care in asking for clarification, commenting, and answering.
Check out our Code of Conduct.
add a comment |
add a comment |
0
active
oldest
votes
Your Answer
StackExchange.ifUsing("editor", function () {
return StackExchange.using("mathjaxEditing", function () {
StackExchange.MarkdownEditor.creationCallbacks.add(function (editor, postfix) {
StackExchange.mathjaxEditing.prepareWmdForMathJax(editor, postfix, [["$", "$"], ["\\(","\\)"]]);
});
});
}, "mathjax-editing");
StackExchange.ready(function() {
var channelOptions = {
tags: "".split(" "),
id: "557"
};
initTagRenderer("".split(" "), "".split(" "), channelOptions);
StackExchange.using("externalEditor", function() {
// Have to fire editor after snippets, if snippets enabled
if (StackExchange.settings.snippets.snippetsEnabled) {
StackExchange.using("snippets", function() {
createEditor();
});
}
else {
createEditor();
}
});
function createEditor() {
StackExchange.prepareEditor({
heartbeatType: 'answer',
autoActivateHeartbeat: false,
convertImagesToLinks: false,
noModals: true,
showLowRepImageUploadWarning: true,
reputationToPostImages: null,
bindNavPrevention: true,
postfix: "",
imageUploader: {
brandingHtml: "Powered by u003ca class="icon-imgur-white" href="https://imgur.com/"u003eu003c/au003e",
contentPolicyHtml: "User contributions licensed under u003ca href="https://creativecommons.org/licenses/by-sa/3.0/"u003ecc by-sa 3.0 with attribution requiredu003c/au003e u003ca href="https://stackoverflow.com/legal/content-policy"u003e(content policy)u003c/au003e",
allowUrls: true
},
onDemand: true,
discardSelector: ".discard-answer"
,immediatelyShowMarkdownHelp:true
});
}
});
Sehee Park is a new contributor. Be nice, and check out our Code of Conduct.
Sign up or log in
StackExchange.ready(function () {
StackExchange.helpers.onClickDraftSave('#login-link');
});
Sign up using Google
Sign up using Facebook
Sign up using Email and Password
Post as a guest
Required, but never shown
StackExchange.ready(
function () {
StackExchange.openid.initPostLogin('.new-post-login', 'https%3a%2f%2fdatascience.stackexchange.com%2fquestions%2f48484%2fvanishing-gradient-problem-for-recent-stochastic-recurrent-neural-networks%23new-answer', 'question_page');
}
);
Post as a guest
Required, but never shown
0
active
oldest
votes
0
active
oldest
votes
active
oldest
votes
active
oldest
votes
Sehee Park is a new contributor. Be nice, and check out our Code of Conduct.
Sehee Park is a new contributor. Be nice, and check out our Code of Conduct.
Sehee Park is a new contributor. Be nice, and check out our Code of Conduct.
Sehee Park is a new contributor. Be nice, and check out our Code of Conduct.
Thanks for contributing an answer to Data Science Stack Exchange!
- Please be sure to answer the question. Provide details and share your research!
But avoid …
- Asking for help, clarification, or responding to other answers.
- Making statements based on opinion; back them up with references or personal experience.
Use MathJax to format equations. MathJax reference.
To learn more, see our tips on writing great answers.
Sign up or log in
StackExchange.ready(function () {
StackExchange.helpers.onClickDraftSave('#login-link');
});
Sign up using Google
Sign up using Facebook
Sign up using Email and Password
Post as a guest
Required, but never shown
StackExchange.ready(
function () {
StackExchange.openid.initPostLogin('.new-post-login', 'https%3a%2f%2fdatascience.stackexchange.com%2fquestions%2f48484%2fvanishing-gradient-problem-for-recent-stochastic-recurrent-neural-networks%23new-answer', 'question_page');
}
);
Post as a guest
Required, but never shown
Sign up or log in
StackExchange.ready(function () {
StackExchange.helpers.onClickDraftSave('#login-link');
});
Sign up using Google
Sign up using Facebook
Sign up using Email and Password
Post as a guest
Required, but never shown
Sign up or log in
StackExchange.ready(function () {
StackExchange.helpers.onClickDraftSave('#login-link');
});
Sign up using Google
Sign up using Facebook
Sign up using Email and Password
Post as a guest
Required, but never shown
Sign up or log in
StackExchange.ready(function () {
StackExchange.helpers.onClickDraftSave('#login-link');
});
Sign up using Google
Sign up using Facebook
Sign up using Email and Password
Sign up using Google
Sign up using Facebook
Sign up using Email and Password
Post as a guest
Required, but never shown
Required, but never shown
Required, but never shown
Required, but never shown
Required, but never shown
Required, but never shown
Required, but never shown
Required, but never shown
Required, but never shown
mx,D,mhu8odRgQA,f,gmZhb7 pJb9Jah8Fn0 zvGm