Performance of model in production varying greatly from train-test data
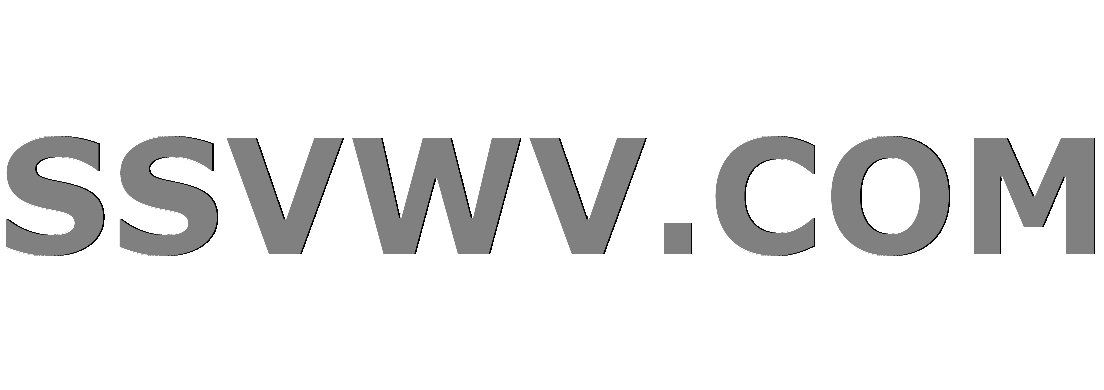
Multi tool use
$begingroup$
I was wondering if anyone has any advice on where to start digging for this problem. I have a model which has gone through development and all train/cv/test data sets now perform above 95% both for accuracy and F-Score. The total development data set is around 60k samples, with a 2/3 split for positive and negative samples. These samples are based on extracts for the months of Jan to Nov of last year. Final test results were:
Precision: 0.9751 Recall: 0.9320 Accuracy 0.9693 F score 0.9531
However, the first runs in production showed a very high precision:95%+ but a very low recall:~50%. Accuracy = 48%, FScore = 68%.
Any thoughts from the group on this, where to look, potential causes. We will run this over the next couple of months, as we may have exceptional variations due to the Xmas period, but we were surprised. Any help appreciated. Thanks
machine-learning supervised-learning accuracy
$endgroup$
bumped to the homepage by Community♦ 3 hours ago
This question has answers that may be good or bad; the system has marked it active so that they can be reviewed.
add a comment |
$begingroup$
I was wondering if anyone has any advice on where to start digging for this problem. I have a model which has gone through development and all train/cv/test data sets now perform above 95% both for accuracy and F-Score. The total development data set is around 60k samples, with a 2/3 split for positive and negative samples. These samples are based on extracts for the months of Jan to Nov of last year. Final test results were:
Precision: 0.9751 Recall: 0.9320 Accuracy 0.9693 F score 0.9531
However, the first runs in production showed a very high precision:95%+ but a very low recall:~50%. Accuracy = 48%, FScore = 68%.
Any thoughts from the group on this, where to look, potential causes. We will run this over the next couple of months, as we may have exceptional variations due to the Xmas period, but we were surprised. Any help appreciated. Thanks
machine-learning supervised-learning accuracy
$endgroup$
bumped to the homepage by Community♦ 3 hours ago
This question has answers that may be good or bad; the system has marked it active so that they can be reviewed.
$begingroup$
Could be way too many things... overfitting? biased sample of training data? wrong train/test/CV process? you didn't measure recall on your test set, nor F-score on your production data, but are trying to compare them.
$endgroup$
– Sean Owen♦
Jan 9 at 16:09
$begingroup$
I have made some changes, hopefully this might give some ideas. During test we had a lot of problems with overfitting, which we finally managed with a combination of dropout, and feature enhancement. With a recall problem we normally look to features, and in which case this maybe consistent with the past. But looking to any other suggestions as well
$endgroup$
– 1961DarthVader
Jan 9 at 16:40
$begingroup$
The most likely explanation is that you have over-fit, and real data from December doesn't really follow the distribution of data from Jan - Nov.
$endgroup$
– Sean Owen♦
Jan 9 at 22:03
$begingroup$
December is a strange month, so while we debug further, we will let the application run thru Jan. Thanks
$endgroup$
– 1961DarthVader
Jan 10 at 6:25
add a comment |
$begingroup$
I was wondering if anyone has any advice on where to start digging for this problem. I have a model which has gone through development and all train/cv/test data sets now perform above 95% both for accuracy and F-Score. The total development data set is around 60k samples, with a 2/3 split for positive and negative samples. These samples are based on extracts for the months of Jan to Nov of last year. Final test results were:
Precision: 0.9751 Recall: 0.9320 Accuracy 0.9693 F score 0.9531
However, the first runs in production showed a very high precision:95%+ but a very low recall:~50%. Accuracy = 48%, FScore = 68%.
Any thoughts from the group on this, where to look, potential causes. We will run this over the next couple of months, as we may have exceptional variations due to the Xmas period, but we were surprised. Any help appreciated. Thanks
machine-learning supervised-learning accuracy
$endgroup$
I was wondering if anyone has any advice on where to start digging for this problem. I have a model which has gone through development and all train/cv/test data sets now perform above 95% both for accuracy and F-Score. The total development data set is around 60k samples, with a 2/3 split for positive and negative samples. These samples are based on extracts for the months of Jan to Nov of last year. Final test results were:
Precision: 0.9751 Recall: 0.9320 Accuracy 0.9693 F score 0.9531
However, the first runs in production showed a very high precision:95%+ but a very low recall:~50%. Accuracy = 48%, FScore = 68%.
Any thoughts from the group on this, where to look, potential causes. We will run this over the next couple of months, as we may have exceptional variations due to the Xmas period, but we were surprised. Any help appreciated. Thanks
machine-learning supervised-learning accuracy
machine-learning supervised-learning accuracy
edited Jan 9 at 16:36
1961DarthVader
asked Jan 9 at 15:50
1961DarthVader1961DarthVader
13
13
bumped to the homepage by Community♦ 3 hours ago
This question has answers that may be good or bad; the system has marked it active so that they can be reviewed.
bumped to the homepage by Community♦ 3 hours ago
This question has answers that may be good or bad; the system has marked it active so that they can be reviewed.
$begingroup$
Could be way too many things... overfitting? biased sample of training data? wrong train/test/CV process? you didn't measure recall on your test set, nor F-score on your production data, but are trying to compare them.
$endgroup$
– Sean Owen♦
Jan 9 at 16:09
$begingroup$
I have made some changes, hopefully this might give some ideas. During test we had a lot of problems with overfitting, which we finally managed with a combination of dropout, and feature enhancement. With a recall problem we normally look to features, and in which case this maybe consistent with the past. But looking to any other suggestions as well
$endgroup$
– 1961DarthVader
Jan 9 at 16:40
$begingroup$
The most likely explanation is that you have over-fit, and real data from December doesn't really follow the distribution of data from Jan - Nov.
$endgroup$
– Sean Owen♦
Jan 9 at 22:03
$begingroup$
December is a strange month, so while we debug further, we will let the application run thru Jan. Thanks
$endgroup$
– 1961DarthVader
Jan 10 at 6:25
add a comment |
$begingroup$
Could be way too many things... overfitting? biased sample of training data? wrong train/test/CV process? you didn't measure recall on your test set, nor F-score on your production data, but are trying to compare them.
$endgroup$
– Sean Owen♦
Jan 9 at 16:09
$begingroup$
I have made some changes, hopefully this might give some ideas. During test we had a lot of problems with overfitting, which we finally managed with a combination of dropout, and feature enhancement. With a recall problem we normally look to features, and in which case this maybe consistent with the past. But looking to any other suggestions as well
$endgroup$
– 1961DarthVader
Jan 9 at 16:40
$begingroup$
The most likely explanation is that you have over-fit, and real data from December doesn't really follow the distribution of data from Jan - Nov.
$endgroup$
– Sean Owen♦
Jan 9 at 22:03
$begingroup$
December is a strange month, so while we debug further, we will let the application run thru Jan. Thanks
$endgroup$
– 1961DarthVader
Jan 10 at 6:25
$begingroup$
Could be way too many things... overfitting? biased sample of training data? wrong train/test/CV process? you didn't measure recall on your test set, nor F-score on your production data, but are trying to compare them.
$endgroup$
– Sean Owen♦
Jan 9 at 16:09
$begingroup$
Could be way too many things... overfitting? biased sample of training data? wrong train/test/CV process? you didn't measure recall on your test set, nor F-score on your production data, but are trying to compare them.
$endgroup$
– Sean Owen♦
Jan 9 at 16:09
$begingroup$
I have made some changes, hopefully this might give some ideas. During test we had a lot of problems with overfitting, which we finally managed with a combination of dropout, and feature enhancement. With a recall problem we normally look to features, and in which case this maybe consistent with the past. But looking to any other suggestions as well
$endgroup$
– 1961DarthVader
Jan 9 at 16:40
$begingroup$
I have made some changes, hopefully this might give some ideas. During test we had a lot of problems with overfitting, which we finally managed with a combination of dropout, and feature enhancement. With a recall problem we normally look to features, and in which case this maybe consistent with the past. But looking to any other suggestions as well
$endgroup$
– 1961DarthVader
Jan 9 at 16:40
$begingroup$
The most likely explanation is that you have over-fit, and real data from December doesn't really follow the distribution of data from Jan - Nov.
$endgroup$
– Sean Owen♦
Jan 9 at 22:03
$begingroup$
The most likely explanation is that you have over-fit, and real data from December doesn't really follow the distribution of data from Jan - Nov.
$endgroup$
– Sean Owen♦
Jan 9 at 22:03
$begingroup$
December is a strange month, so while we debug further, we will let the application run thru Jan. Thanks
$endgroup$
– 1961DarthVader
Jan 10 at 6:25
$begingroup$
December is a strange month, so while we debug further, we will let the application run thru Jan. Thanks
$endgroup$
– 1961DarthVader
Jan 10 at 6:25
add a comment |
1 Answer
1
active
oldest
votes
$begingroup$
This happened to me in the past.
The first question I would ask myself will be, how am I splitting train, validation and test sets? The second one would be, are my production data in the same domain as of those in train/validation/test?
Sometimes a simple split of data might still result in testing data leaking into the validation and even test data, this is the case of domains where we want to study the behaviour of users, normally you will not want the same users in train/test/validation sets (that is, ideally a user should remain within the same data set).
Apart from that, looks like you might just be overfitting the data.
$endgroup$
$begingroup$
Given the trouble we had with overfitting during development, I was hoping that we would not have to go there again, but it it seems a sensible place to start. Also we know December is a strange month, so there could be exceptional data there. While we run other tests, we will let the solution run thru Jan and see how we perform. Thanks
$endgroup$
– 1961DarthVader
Jan 10 at 6:21
add a comment |
Your Answer
StackExchange.ifUsing("editor", function () {
return StackExchange.using("mathjaxEditing", function () {
StackExchange.MarkdownEditor.creationCallbacks.add(function (editor, postfix) {
StackExchange.mathjaxEditing.prepareWmdForMathJax(editor, postfix, [["$", "$"], ["\\(","\\)"]]);
});
});
}, "mathjax-editing");
StackExchange.ready(function() {
var channelOptions = {
tags: "".split(" "),
id: "557"
};
initTagRenderer("".split(" "), "".split(" "), channelOptions);
StackExchange.using("externalEditor", function() {
// Have to fire editor after snippets, if snippets enabled
if (StackExchange.settings.snippets.snippetsEnabled) {
StackExchange.using("snippets", function() {
createEditor();
});
}
else {
createEditor();
}
});
function createEditor() {
StackExchange.prepareEditor({
heartbeatType: 'answer',
autoActivateHeartbeat: false,
convertImagesToLinks: false,
noModals: true,
showLowRepImageUploadWarning: true,
reputationToPostImages: null,
bindNavPrevention: true,
postfix: "",
imageUploader: {
brandingHtml: "Powered by u003ca class="icon-imgur-white" href="https://imgur.com/"u003eu003c/au003e",
contentPolicyHtml: "User contributions licensed under u003ca href="https://creativecommons.org/licenses/by-sa/3.0/"u003ecc by-sa 3.0 with attribution requiredu003c/au003e u003ca href="https://stackoverflow.com/legal/content-policy"u003e(content policy)u003c/au003e",
allowUrls: true
},
onDemand: true,
discardSelector: ".discard-answer"
,immediatelyShowMarkdownHelp:true
});
}
});
Sign up or log in
StackExchange.ready(function () {
StackExchange.helpers.onClickDraftSave('#login-link');
});
Sign up using Google
Sign up using Facebook
Sign up using Email and Password
Post as a guest
Required, but never shown
StackExchange.ready(
function () {
StackExchange.openid.initPostLogin('.new-post-login', 'https%3a%2f%2fdatascience.stackexchange.com%2fquestions%2f43728%2fperformance-of-model-in-production-varying-greatly-from-train-test-data%23new-answer', 'question_page');
}
);
Post as a guest
Required, but never shown
1 Answer
1
active
oldest
votes
1 Answer
1
active
oldest
votes
active
oldest
votes
active
oldest
votes
$begingroup$
This happened to me in the past.
The first question I would ask myself will be, how am I splitting train, validation and test sets? The second one would be, are my production data in the same domain as of those in train/validation/test?
Sometimes a simple split of data might still result in testing data leaking into the validation and even test data, this is the case of domains where we want to study the behaviour of users, normally you will not want the same users in train/test/validation sets (that is, ideally a user should remain within the same data set).
Apart from that, looks like you might just be overfitting the data.
$endgroup$
$begingroup$
Given the trouble we had with overfitting during development, I was hoping that we would not have to go there again, but it it seems a sensible place to start. Also we know December is a strange month, so there could be exceptional data there. While we run other tests, we will let the solution run thru Jan and see how we perform. Thanks
$endgroup$
– 1961DarthVader
Jan 10 at 6:21
add a comment |
$begingroup$
This happened to me in the past.
The first question I would ask myself will be, how am I splitting train, validation and test sets? The second one would be, are my production data in the same domain as of those in train/validation/test?
Sometimes a simple split of data might still result in testing data leaking into the validation and even test data, this is the case of domains where we want to study the behaviour of users, normally you will not want the same users in train/test/validation sets (that is, ideally a user should remain within the same data set).
Apart from that, looks like you might just be overfitting the data.
$endgroup$
$begingroup$
Given the trouble we had with overfitting during development, I was hoping that we would not have to go there again, but it it seems a sensible place to start. Also we know December is a strange month, so there could be exceptional data there. While we run other tests, we will let the solution run thru Jan and see how we perform. Thanks
$endgroup$
– 1961DarthVader
Jan 10 at 6:21
add a comment |
$begingroup$
This happened to me in the past.
The first question I would ask myself will be, how am I splitting train, validation and test sets? The second one would be, are my production data in the same domain as of those in train/validation/test?
Sometimes a simple split of data might still result in testing data leaking into the validation and even test data, this is the case of domains where we want to study the behaviour of users, normally you will not want the same users in train/test/validation sets (that is, ideally a user should remain within the same data set).
Apart from that, looks like you might just be overfitting the data.
$endgroup$
This happened to me in the past.
The first question I would ask myself will be, how am I splitting train, validation and test sets? The second one would be, are my production data in the same domain as of those in train/validation/test?
Sometimes a simple split of data might still result in testing data leaking into the validation and even test data, this is the case of domains where we want to study the behaviour of users, normally you will not want the same users in train/test/validation sets (that is, ideally a user should remain within the same data set).
Apart from that, looks like you might just be overfitting the data.
answered Jan 10 at 2:32
Juan Antonio Gomez MorianoJuan Antonio Gomez Moriano
641213
641213
$begingroup$
Given the trouble we had with overfitting during development, I was hoping that we would not have to go there again, but it it seems a sensible place to start. Also we know December is a strange month, so there could be exceptional data there. While we run other tests, we will let the solution run thru Jan and see how we perform. Thanks
$endgroup$
– 1961DarthVader
Jan 10 at 6:21
add a comment |
$begingroup$
Given the trouble we had with overfitting during development, I was hoping that we would not have to go there again, but it it seems a sensible place to start. Also we know December is a strange month, so there could be exceptional data there. While we run other tests, we will let the solution run thru Jan and see how we perform. Thanks
$endgroup$
– 1961DarthVader
Jan 10 at 6:21
$begingroup$
Given the trouble we had with overfitting during development, I was hoping that we would not have to go there again, but it it seems a sensible place to start. Also we know December is a strange month, so there could be exceptional data there. While we run other tests, we will let the solution run thru Jan and see how we perform. Thanks
$endgroup$
– 1961DarthVader
Jan 10 at 6:21
$begingroup$
Given the trouble we had with overfitting during development, I was hoping that we would not have to go there again, but it it seems a sensible place to start. Also we know December is a strange month, so there could be exceptional data there. While we run other tests, we will let the solution run thru Jan and see how we perform. Thanks
$endgroup$
– 1961DarthVader
Jan 10 at 6:21
add a comment |
Thanks for contributing an answer to Data Science Stack Exchange!
- Please be sure to answer the question. Provide details and share your research!
But avoid …
- Asking for help, clarification, or responding to other answers.
- Making statements based on opinion; back them up with references or personal experience.
Use MathJax to format equations. MathJax reference.
To learn more, see our tips on writing great answers.
Sign up or log in
StackExchange.ready(function () {
StackExchange.helpers.onClickDraftSave('#login-link');
});
Sign up using Google
Sign up using Facebook
Sign up using Email and Password
Post as a guest
Required, but never shown
StackExchange.ready(
function () {
StackExchange.openid.initPostLogin('.new-post-login', 'https%3a%2f%2fdatascience.stackexchange.com%2fquestions%2f43728%2fperformance-of-model-in-production-varying-greatly-from-train-test-data%23new-answer', 'question_page');
}
);
Post as a guest
Required, but never shown
Sign up or log in
StackExchange.ready(function () {
StackExchange.helpers.onClickDraftSave('#login-link');
});
Sign up using Google
Sign up using Facebook
Sign up using Email and Password
Post as a guest
Required, but never shown
Sign up or log in
StackExchange.ready(function () {
StackExchange.helpers.onClickDraftSave('#login-link');
});
Sign up using Google
Sign up using Facebook
Sign up using Email and Password
Post as a guest
Required, but never shown
Sign up or log in
StackExchange.ready(function () {
StackExchange.helpers.onClickDraftSave('#login-link');
});
Sign up using Google
Sign up using Facebook
Sign up using Email and Password
Sign up using Google
Sign up using Facebook
Sign up using Email and Password
Post as a guest
Required, but never shown
Required, but never shown
Required, but never shown
Required, but never shown
Required, but never shown
Required, but never shown
Required, but never shown
Required, but never shown
Required, but never shown
FEQqoK2HsArlW gZn6FS4IeUW3g8E9db7
$begingroup$
Could be way too many things... overfitting? biased sample of training data? wrong train/test/CV process? you didn't measure recall on your test set, nor F-score on your production data, but are trying to compare them.
$endgroup$
– Sean Owen♦
Jan 9 at 16:09
$begingroup$
I have made some changes, hopefully this might give some ideas. During test we had a lot of problems with overfitting, which we finally managed with a combination of dropout, and feature enhancement. With a recall problem we normally look to features, and in which case this maybe consistent with the past. But looking to any other suggestions as well
$endgroup$
– 1961DarthVader
Jan 9 at 16:40
$begingroup$
The most likely explanation is that you have over-fit, and real data from December doesn't really follow the distribution of data from Jan - Nov.
$endgroup$
– Sean Owen♦
Jan 9 at 22:03
$begingroup$
December is a strange month, so while we debug further, we will let the application run thru Jan. Thanks
$endgroup$
– 1961DarthVader
Jan 10 at 6:25