Strategies for time series forecasting for 2000 different products?
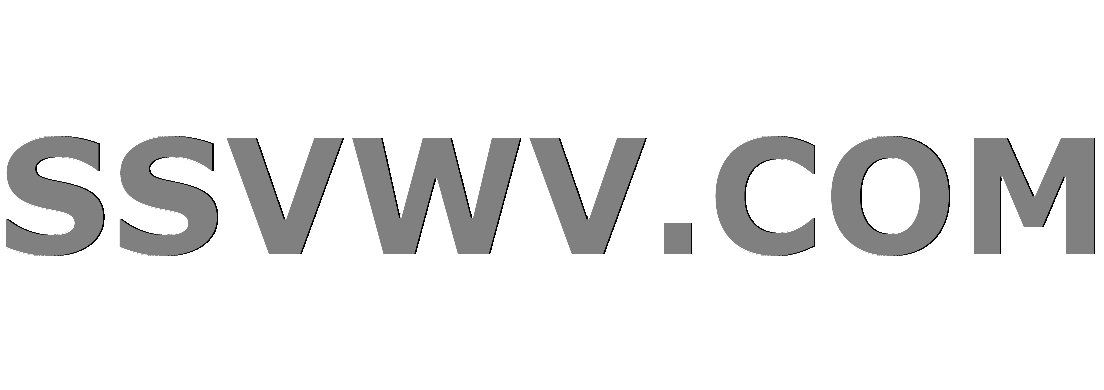
Multi tool use
$begingroup$
First of all, I realise that my question is very broad and that it may be hard to answer this question because of it.
Do you have any advice on how to approach a 'problem' where you need to make forecasts/predictions for 2000+ different products? In other words, each product requires a different forecast/prediction. I have 2 years of historical data on week level (i.e. demand per week per product).
I need to do this in a short time period: I have about a week to do this, hence I am looking for ways that I can quickly make relatively good prediction models. Creating a model for each product and inspecting its performance closely, one by one, would be too time-consuming.
I thought of segmenting the products based on the variance, so that I can employ simple models for products that have a low variance. While this is probably not ideal, it would be a quick way to narrow down the number of models I need to create.
It would be greatly appreciated if you have any practical advice for me on approaching this problem.
time-series forecasting segmentation
$endgroup$
add a comment |
$begingroup$
First of all, I realise that my question is very broad and that it may be hard to answer this question because of it.
Do you have any advice on how to approach a 'problem' where you need to make forecasts/predictions for 2000+ different products? In other words, each product requires a different forecast/prediction. I have 2 years of historical data on week level (i.e. demand per week per product).
I need to do this in a short time period: I have about a week to do this, hence I am looking for ways that I can quickly make relatively good prediction models. Creating a model for each product and inspecting its performance closely, one by one, would be too time-consuming.
I thought of segmenting the products based on the variance, so that I can employ simple models for products that have a low variance. While this is probably not ideal, it would be a quick way to narrow down the number of models I need to create.
It would be greatly appreciated if you have any practical advice for me on approaching this problem.
time-series forecasting segmentation
$endgroup$
$begingroup$
Are those similar products? You might benefit from searching this sitr for hierarchical forecasting
$endgroup$
– kjetil b halvorsen
4 hours ago
add a comment |
$begingroup$
First of all, I realise that my question is very broad and that it may be hard to answer this question because of it.
Do you have any advice on how to approach a 'problem' where you need to make forecasts/predictions for 2000+ different products? In other words, each product requires a different forecast/prediction. I have 2 years of historical data on week level (i.e. demand per week per product).
I need to do this in a short time period: I have about a week to do this, hence I am looking for ways that I can quickly make relatively good prediction models. Creating a model for each product and inspecting its performance closely, one by one, would be too time-consuming.
I thought of segmenting the products based on the variance, so that I can employ simple models for products that have a low variance. While this is probably not ideal, it would be a quick way to narrow down the number of models I need to create.
It would be greatly appreciated if you have any practical advice for me on approaching this problem.
time-series forecasting segmentation
$endgroup$
First of all, I realise that my question is very broad and that it may be hard to answer this question because of it.
Do you have any advice on how to approach a 'problem' where you need to make forecasts/predictions for 2000+ different products? In other words, each product requires a different forecast/prediction. I have 2 years of historical data on week level (i.e. demand per week per product).
I need to do this in a short time period: I have about a week to do this, hence I am looking for ways that I can quickly make relatively good prediction models. Creating a model for each product and inspecting its performance closely, one by one, would be too time-consuming.
I thought of segmenting the products based on the variance, so that I can employ simple models for products that have a low variance. While this is probably not ideal, it would be a quick way to narrow down the number of models I need to create.
It would be greatly appreciated if you have any practical advice for me on approaching this problem.
time-series forecasting segmentation
time-series forecasting segmentation
asked 5 hours ago
AmonetAmonet
17012
17012
$begingroup$
Are those similar products? You might benefit from searching this sitr for hierarchical forecasting
$endgroup$
– kjetil b halvorsen
4 hours ago
add a comment |
$begingroup$
Are those similar products? You might benefit from searching this sitr for hierarchical forecasting
$endgroup$
– kjetil b halvorsen
4 hours ago
$begingroup$
Are those similar products? You might benefit from searching this sitr for hierarchical forecasting
$endgroup$
– kjetil b halvorsen
4 hours ago
$begingroup$
Are those similar products? You might benefit from searching this sitr for hierarchical forecasting
$endgroup$
– kjetil b halvorsen
4 hours ago
add a comment |
3 Answers
3
active
oldest
votes
$begingroup$
We will only be able to give you very general advice.
- Are there any strong drivers, like promotions or calendar events, or seasonality, trends or lifecycles? If so, include them in your models. For instance, you could regress sales on promotions, then potentially model residuals (using exponential smoothing or ARIMA).
- There are software packages that do a reasonably good job at fitting multiple time series models to a series. You can then simply iterate over your 2000 series, which should not take much more runtime than a cup of coffee. I particularly recommend the
ets()
function in theforecast
package in R. (Less so theauto.arima()
function for weekly data. - At least skim a forecasting textbook, e.g., this one. It uses the
forecast
package I recommend above. - What is your final objective? Do you want an unbiased forecast? Then assess point forecasts using the MSE. Will your bonus depend on the MAPE? Then this list of the problems of the MAPE may be helpful. Do you need forecasts to set safety amounts? Then you need quantile forecasts, not mean predictions. (The functions in the
forecast
package can give you those.)
If you have more specific questions, do post them at CV.
$endgroup$
$begingroup$
I'm sorry for this little off-topic question, but what material on forecasting (in general) would you recommend after studying 'Forecting: Principles and Practice' you mention? :-)
$endgroup$
– Łukasz Grad
5 hours ago
3
$begingroup$
@ŁukaszGrad: if you have worked your way through FPP2, our book won't tell you much new. Ord et al.'s Principles of Business Forecasting (2nd ed.) goes into more depth (I reviewed it here if you have access). ...
$endgroup$
– Stephan Kolassa
5 hours ago
2
$begingroup$
... You might profit from looking at the IIF, maybe read its publication Foresight or attend one of its conferences, either the ISF, which will take place this year in June in Thessaloniki, or the Foresight Practitioner Conference, this year in November at the SAS campus in Cary, NC, depending on where you are. The ISF is somewhat more academically oriented, but recently, I'd say about 33% of attendees came from industry, and there usually is a practitioner track.
$endgroup$
– Stephan Kolassa
5 hours ago
1
$begingroup$
(Full disclosure: I am involved with all of these, so take my recommendations with a large grain of salt. If you do attend one of the conferences, find me and say hi!)
$endgroup$
– Stephan Kolassa
5 hours ago
$begingroup$
Thank you for all this detailed information! I must say I discovered FPP2 through one of your other posts here on CV and I think it is a great book. Forecasting is something I neglected in the past :-)
$endgroup$
– Łukasz Grad
4 hours ago
|
show 2 more comments
$begingroup$
A follow up to @StephanKolassa 's answer:
I concur with Prof Kolassa that ETS() from the forecast package in R is probably your best and fastest choice. If ETS doesn't give good results, you might want also want to use Facebook's Prophet package (Auto.arima is easy to use, but two years of weekly data is bordering not enough data for an ARIMA model in my experience). Personally I have found Prophet to be easier to user when you have promotions and holiday event data available, otherwise ETS() might work better. Your real challenge is more of a coding challenge of how to efficiently iterate it over a large number of time series. You can check this response for more details on how to automate forecast generation.
In demand forecasting, some form of hierarchical forecasting is frequently performed. I.e you have 2000 products and you need a separate forecast for each separate product, but there are similarities between products that might help with the forecasting. You want to find some way of grouping the product together along a product hierarchy and then use hierarchical forecasting to improve accuracy. Since you are looking for forecasts at the individual product level, look at trying the top-down hierarchical approach.
Something a little bit more farfetched, but I would like call it out: Amazon and Uber use neural networks for this type of problem, where instead of having a separate forecast for each product/time series, they use one gigantic recurrent neural network to forecast all the time series in bulk. Note that they still end up with individual forecasts for each product (in Uber's case it is traffic/demand per city as opposed to products), they are just using a large model (an LSTM deep learning model) to do it all at once. The idea is similar in spirit to hierarchical forecasting in the sense that the neural network learns from the similarities between the histories of different products to come up with better forecasts. The Uber team has made some of their code available (through the M4 competition Github repositories), however it is C++ code (not exactly the favorite language of the stats crowd). Amazon's approach is not open source and you have to use their paid Amazon Forecast service to do the forecasts.
$endgroup$
add a comment |
$begingroup$
Segmenting based on the variance of the original series makes no sense to me as the best model should be invariant to scale. Consider a series ..model it and then multiply each value in the time series by 1000 .
In terms of mass producing equations that may have both deterministic structure (pulses/level shift/local time trends ) OR either auto-regressive seasonality and arima structure you have to run a computer-based script . Beware of simple auto arima solutions that assume no deterministic structure OR fixed assumptions about same.
$endgroup$
add a comment |
Your Answer
StackExchange.ifUsing("editor", function () {
return StackExchange.using("mathjaxEditing", function () {
StackExchange.MarkdownEditor.creationCallbacks.add(function (editor, postfix) {
StackExchange.mathjaxEditing.prepareWmdForMathJax(editor, postfix, [["$", "$"], ["\\(","\\)"]]);
});
});
}, "mathjax-editing");
StackExchange.ready(function() {
var channelOptions = {
tags: "".split(" "),
id: "65"
};
initTagRenderer("".split(" "), "".split(" "), channelOptions);
StackExchange.using("externalEditor", function() {
// Have to fire editor after snippets, if snippets enabled
if (StackExchange.settings.snippets.snippetsEnabled) {
StackExchange.using("snippets", function() {
createEditor();
});
}
else {
createEditor();
}
});
function createEditor() {
StackExchange.prepareEditor({
heartbeatType: 'answer',
autoActivateHeartbeat: false,
convertImagesToLinks: false,
noModals: true,
showLowRepImageUploadWarning: true,
reputationToPostImages: null,
bindNavPrevention: true,
postfix: "",
imageUploader: {
brandingHtml: "Powered by u003ca class="icon-imgur-white" href="https://imgur.com/"u003eu003c/au003e",
contentPolicyHtml: "User contributions licensed under u003ca href="https://creativecommons.org/licenses/by-sa/3.0/"u003ecc by-sa 3.0 with attribution requiredu003c/au003e u003ca href="https://stackoverflow.com/legal/content-policy"u003e(content policy)u003c/au003e",
allowUrls: true
},
onDemand: true,
discardSelector: ".discard-answer"
,immediatelyShowMarkdownHelp:true
});
}
});
Sign up or log in
StackExchange.ready(function () {
StackExchange.helpers.onClickDraftSave('#login-link');
});
Sign up using Google
Sign up using Facebook
Sign up using Email and Password
Post as a guest
Required, but never shown
StackExchange.ready(
function () {
StackExchange.openid.initPostLogin('.new-post-login', 'https%3a%2f%2fstats.stackexchange.com%2fquestions%2f389291%2fstrategies-for-time-series-forecasting-for-2000-different-products%23new-answer', 'question_page');
}
);
Post as a guest
Required, but never shown
3 Answers
3
active
oldest
votes
3 Answers
3
active
oldest
votes
active
oldest
votes
active
oldest
votes
$begingroup$
We will only be able to give you very general advice.
- Are there any strong drivers, like promotions or calendar events, or seasonality, trends or lifecycles? If so, include them in your models. For instance, you could regress sales on promotions, then potentially model residuals (using exponential smoothing or ARIMA).
- There are software packages that do a reasonably good job at fitting multiple time series models to a series. You can then simply iterate over your 2000 series, which should not take much more runtime than a cup of coffee. I particularly recommend the
ets()
function in theforecast
package in R. (Less so theauto.arima()
function for weekly data. - At least skim a forecasting textbook, e.g., this one. It uses the
forecast
package I recommend above. - What is your final objective? Do you want an unbiased forecast? Then assess point forecasts using the MSE. Will your bonus depend on the MAPE? Then this list of the problems of the MAPE may be helpful. Do you need forecasts to set safety amounts? Then you need quantile forecasts, not mean predictions. (The functions in the
forecast
package can give you those.)
If you have more specific questions, do post them at CV.
$endgroup$
$begingroup$
I'm sorry for this little off-topic question, but what material on forecasting (in general) would you recommend after studying 'Forecting: Principles and Practice' you mention? :-)
$endgroup$
– Łukasz Grad
5 hours ago
3
$begingroup$
@ŁukaszGrad: if you have worked your way through FPP2, our book won't tell you much new. Ord et al.'s Principles of Business Forecasting (2nd ed.) goes into more depth (I reviewed it here if you have access). ...
$endgroup$
– Stephan Kolassa
5 hours ago
2
$begingroup$
... You might profit from looking at the IIF, maybe read its publication Foresight or attend one of its conferences, either the ISF, which will take place this year in June in Thessaloniki, or the Foresight Practitioner Conference, this year in November at the SAS campus in Cary, NC, depending on where you are. The ISF is somewhat more academically oriented, but recently, I'd say about 33% of attendees came from industry, and there usually is a practitioner track.
$endgroup$
– Stephan Kolassa
5 hours ago
1
$begingroup$
(Full disclosure: I am involved with all of these, so take my recommendations with a large grain of salt. If you do attend one of the conferences, find me and say hi!)
$endgroup$
– Stephan Kolassa
5 hours ago
$begingroup$
Thank you for all this detailed information! I must say I discovered FPP2 through one of your other posts here on CV and I think it is a great book. Forecasting is something I neglected in the past :-)
$endgroup$
– Łukasz Grad
4 hours ago
|
show 2 more comments
$begingroup$
We will only be able to give you very general advice.
- Are there any strong drivers, like promotions or calendar events, or seasonality, trends or lifecycles? If so, include them in your models. For instance, you could regress sales on promotions, then potentially model residuals (using exponential smoothing or ARIMA).
- There are software packages that do a reasonably good job at fitting multiple time series models to a series. You can then simply iterate over your 2000 series, which should not take much more runtime than a cup of coffee. I particularly recommend the
ets()
function in theforecast
package in R. (Less so theauto.arima()
function for weekly data. - At least skim a forecasting textbook, e.g., this one. It uses the
forecast
package I recommend above. - What is your final objective? Do you want an unbiased forecast? Then assess point forecasts using the MSE. Will your bonus depend on the MAPE? Then this list of the problems of the MAPE may be helpful. Do you need forecasts to set safety amounts? Then you need quantile forecasts, not mean predictions. (The functions in the
forecast
package can give you those.)
If you have more specific questions, do post them at CV.
$endgroup$
$begingroup$
I'm sorry for this little off-topic question, but what material on forecasting (in general) would you recommend after studying 'Forecting: Principles and Practice' you mention? :-)
$endgroup$
– Łukasz Grad
5 hours ago
3
$begingroup$
@ŁukaszGrad: if you have worked your way through FPP2, our book won't tell you much new. Ord et al.'s Principles of Business Forecasting (2nd ed.) goes into more depth (I reviewed it here if you have access). ...
$endgroup$
– Stephan Kolassa
5 hours ago
2
$begingroup$
... You might profit from looking at the IIF, maybe read its publication Foresight or attend one of its conferences, either the ISF, which will take place this year in June in Thessaloniki, or the Foresight Practitioner Conference, this year in November at the SAS campus in Cary, NC, depending on where you are. The ISF is somewhat more academically oriented, but recently, I'd say about 33% of attendees came from industry, and there usually is a practitioner track.
$endgroup$
– Stephan Kolassa
5 hours ago
1
$begingroup$
(Full disclosure: I am involved with all of these, so take my recommendations with a large grain of salt. If you do attend one of the conferences, find me and say hi!)
$endgroup$
– Stephan Kolassa
5 hours ago
$begingroup$
Thank you for all this detailed information! I must say I discovered FPP2 through one of your other posts here on CV and I think it is a great book. Forecasting is something I neglected in the past :-)
$endgroup$
– Łukasz Grad
4 hours ago
|
show 2 more comments
$begingroup$
We will only be able to give you very general advice.
- Are there any strong drivers, like promotions or calendar events, or seasonality, trends or lifecycles? If so, include them in your models. For instance, you could regress sales on promotions, then potentially model residuals (using exponential smoothing or ARIMA).
- There are software packages that do a reasonably good job at fitting multiple time series models to a series. You can then simply iterate over your 2000 series, which should not take much more runtime than a cup of coffee. I particularly recommend the
ets()
function in theforecast
package in R. (Less so theauto.arima()
function for weekly data. - At least skim a forecasting textbook, e.g., this one. It uses the
forecast
package I recommend above. - What is your final objective? Do you want an unbiased forecast? Then assess point forecasts using the MSE. Will your bonus depend on the MAPE? Then this list of the problems of the MAPE may be helpful. Do you need forecasts to set safety amounts? Then you need quantile forecasts, not mean predictions. (The functions in the
forecast
package can give you those.)
If you have more specific questions, do post them at CV.
$endgroup$
We will only be able to give you very general advice.
- Are there any strong drivers, like promotions or calendar events, or seasonality, trends or lifecycles? If so, include them in your models. For instance, you could regress sales on promotions, then potentially model residuals (using exponential smoothing or ARIMA).
- There are software packages that do a reasonably good job at fitting multiple time series models to a series. You can then simply iterate over your 2000 series, which should not take much more runtime than a cup of coffee. I particularly recommend the
ets()
function in theforecast
package in R. (Less so theauto.arima()
function for weekly data. - At least skim a forecasting textbook, e.g., this one. It uses the
forecast
package I recommend above. - What is your final objective? Do you want an unbiased forecast? Then assess point forecasts using the MSE. Will your bonus depend on the MAPE? Then this list of the problems of the MAPE may be helpful. Do you need forecasts to set safety amounts? Then you need quantile forecasts, not mean predictions. (The functions in the
forecast
package can give you those.)
If you have more specific questions, do post them at CV.
answered 5 hours ago
Stephan KolassaStephan Kolassa
44.6k693163
44.6k693163
$begingroup$
I'm sorry for this little off-topic question, but what material on forecasting (in general) would you recommend after studying 'Forecting: Principles and Practice' you mention? :-)
$endgroup$
– Łukasz Grad
5 hours ago
3
$begingroup$
@ŁukaszGrad: if you have worked your way through FPP2, our book won't tell you much new. Ord et al.'s Principles of Business Forecasting (2nd ed.) goes into more depth (I reviewed it here if you have access). ...
$endgroup$
– Stephan Kolassa
5 hours ago
2
$begingroup$
... You might profit from looking at the IIF, maybe read its publication Foresight or attend one of its conferences, either the ISF, which will take place this year in June in Thessaloniki, or the Foresight Practitioner Conference, this year in November at the SAS campus in Cary, NC, depending on where you are. The ISF is somewhat more academically oriented, but recently, I'd say about 33% of attendees came from industry, and there usually is a practitioner track.
$endgroup$
– Stephan Kolassa
5 hours ago
1
$begingroup$
(Full disclosure: I am involved with all of these, so take my recommendations with a large grain of salt. If you do attend one of the conferences, find me and say hi!)
$endgroup$
– Stephan Kolassa
5 hours ago
$begingroup$
Thank you for all this detailed information! I must say I discovered FPP2 through one of your other posts here on CV and I think it is a great book. Forecasting is something I neglected in the past :-)
$endgroup$
– Łukasz Grad
4 hours ago
|
show 2 more comments
$begingroup$
I'm sorry for this little off-topic question, but what material on forecasting (in general) would you recommend after studying 'Forecting: Principles and Practice' you mention? :-)
$endgroup$
– Łukasz Grad
5 hours ago
3
$begingroup$
@ŁukaszGrad: if you have worked your way through FPP2, our book won't tell you much new. Ord et al.'s Principles of Business Forecasting (2nd ed.) goes into more depth (I reviewed it here if you have access). ...
$endgroup$
– Stephan Kolassa
5 hours ago
2
$begingroup$
... You might profit from looking at the IIF, maybe read its publication Foresight or attend one of its conferences, either the ISF, which will take place this year in June in Thessaloniki, or the Foresight Practitioner Conference, this year in November at the SAS campus in Cary, NC, depending on where you are. The ISF is somewhat more academically oriented, but recently, I'd say about 33% of attendees came from industry, and there usually is a practitioner track.
$endgroup$
– Stephan Kolassa
5 hours ago
1
$begingroup$
(Full disclosure: I am involved with all of these, so take my recommendations with a large grain of salt. If you do attend one of the conferences, find me and say hi!)
$endgroup$
– Stephan Kolassa
5 hours ago
$begingroup$
Thank you for all this detailed information! I must say I discovered FPP2 through one of your other posts here on CV and I think it is a great book. Forecasting is something I neglected in the past :-)
$endgroup$
– Łukasz Grad
4 hours ago
$begingroup$
I'm sorry for this little off-topic question, but what material on forecasting (in general) would you recommend after studying 'Forecting: Principles and Practice' you mention? :-)
$endgroup$
– Łukasz Grad
5 hours ago
$begingroup$
I'm sorry for this little off-topic question, but what material on forecasting (in general) would you recommend after studying 'Forecting: Principles and Practice' you mention? :-)
$endgroup$
– Łukasz Grad
5 hours ago
3
3
$begingroup$
@ŁukaszGrad: if you have worked your way through FPP2, our book won't tell you much new. Ord et al.'s Principles of Business Forecasting (2nd ed.) goes into more depth (I reviewed it here if you have access). ...
$endgroup$
– Stephan Kolassa
5 hours ago
$begingroup$
@ŁukaszGrad: if you have worked your way through FPP2, our book won't tell you much new. Ord et al.'s Principles of Business Forecasting (2nd ed.) goes into more depth (I reviewed it here if you have access). ...
$endgroup$
– Stephan Kolassa
5 hours ago
2
2
$begingroup$
... You might profit from looking at the IIF, maybe read its publication Foresight or attend one of its conferences, either the ISF, which will take place this year in June in Thessaloniki, or the Foresight Practitioner Conference, this year in November at the SAS campus in Cary, NC, depending on where you are. The ISF is somewhat more academically oriented, but recently, I'd say about 33% of attendees came from industry, and there usually is a practitioner track.
$endgroup$
– Stephan Kolassa
5 hours ago
$begingroup$
... You might profit from looking at the IIF, maybe read its publication Foresight or attend one of its conferences, either the ISF, which will take place this year in June in Thessaloniki, or the Foresight Practitioner Conference, this year in November at the SAS campus in Cary, NC, depending on where you are. The ISF is somewhat more academically oriented, but recently, I'd say about 33% of attendees came from industry, and there usually is a practitioner track.
$endgroup$
– Stephan Kolassa
5 hours ago
1
1
$begingroup$
(Full disclosure: I am involved with all of these, so take my recommendations with a large grain of salt. If you do attend one of the conferences, find me and say hi!)
$endgroup$
– Stephan Kolassa
5 hours ago
$begingroup$
(Full disclosure: I am involved with all of these, so take my recommendations with a large grain of salt. If you do attend one of the conferences, find me and say hi!)
$endgroup$
– Stephan Kolassa
5 hours ago
$begingroup$
Thank you for all this detailed information! I must say I discovered FPP2 through one of your other posts here on CV and I think it is a great book. Forecasting is something I neglected in the past :-)
$endgroup$
– Łukasz Grad
4 hours ago
$begingroup$
Thank you for all this detailed information! I must say I discovered FPP2 through one of your other posts here on CV and I think it is a great book. Forecasting is something I neglected in the past :-)
$endgroup$
– Łukasz Grad
4 hours ago
|
show 2 more comments
$begingroup$
A follow up to @StephanKolassa 's answer:
I concur with Prof Kolassa that ETS() from the forecast package in R is probably your best and fastest choice. If ETS doesn't give good results, you might want also want to use Facebook's Prophet package (Auto.arima is easy to use, but two years of weekly data is bordering not enough data for an ARIMA model in my experience). Personally I have found Prophet to be easier to user when you have promotions and holiday event data available, otherwise ETS() might work better. Your real challenge is more of a coding challenge of how to efficiently iterate it over a large number of time series. You can check this response for more details on how to automate forecast generation.
In demand forecasting, some form of hierarchical forecasting is frequently performed. I.e you have 2000 products and you need a separate forecast for each separate product, but there are similarities between products that might help with the forecasting. You want to find some way of grouping the product together along a product hierarchy and then use hierarchical forecasting to improve accuracy. Since you are looking for forecasts at the individual product level, look at trying the top-down hierarchical approach.
Something a little bit more farfetched, but I would like call it out: Amazon and Uber use neural networks for this type of problem, where instead of having a separate forecast for each product/time series, they use one gigantic recurrent neural network to forecast all the time series in bulk. Note that they still end up with individual forecasts for each product (in Uber's case it is traffic/demand per city as opposed to products), they are just using a large model (an LSTM deep learning model) to do it all at once. The idea is similar in spirit to hierarchical forecasting in the sense that the neural network learns from the similarities between the histories of different products to come up with better forecasts. The Uber team has made some of their code available (through the M4 competition Github repositories), however it is C++ code (not exactly the favorite language of the stats crowd). Amazon's approach is not open source and you have to use their paid Amazon Forecast service to do the forecasts.
$endgroup$
add a comment |
$begingroup$
A follow up to @StephanKolassa 's answer:
I concur with Prof Kolassa that ETS() from the forecast package in R is probably your best and fastest choice. If ETS doesn't give good results, you might want also want to use Facebook's Prophet package (Auto.arima is easy to use, but two years of weekly data is bordering not enough data for an ARIMA model in my experience). Personally I have found Prophet to be easier to user when you have promotions and holiday event data available, otherwise ETS() might work better. Your real challenge is more of a coding challenge of how to efficiently iterate it over a large number of time series. You can check this response for more details on how to automate forecast generation.
In demand forecasting, some form of hierarchical forecasting is frequently performed. I.e you have 2000 products and you need a separate forecast for each separate product, but there are similarities between products that might help with the forecasting. You want to find some way of grouping the product together along a product hierarchy and then use hierarchical forecasting to improve accuracy. Since you are looking for forecasts at the individual product level, look at trying the top-down hierarchical approach.
Something a little bit more farfetched, but I would like call it out: Amazon and Uber use neural networks for this type of problem, where instead of having a separate forecast for each product/time series, they use one gigantic recurrent neural network to forecast all the time series in bulk. Note that they still end up with individual forecasts for each product (in Uber's case it is traffic/demand per city as opposed to products), they are just using a large model (an LSTM deep learning model) to do it all at once. The idea is similar in spirit to hierarchical forecasting in the sense that the neural network learns from the similarities between the histories of different products to come up with better forecasts. The Uber team has made some of their code available (through the M4 competition Github repositories), however it is C++ code (not exactly the favorite language of the stats crowd). Amazon's approach is not open source and you have to use their paid Amazon Forecast service to do the forecasts.
$endgroup$
add a comment |
$begingroup$
A follow up to @StephanKolassa 's answer:
I concur with Prof Kolassa that ETS() from the forecast package in R is probably your best and fastest choice. If ETS doesn't give good results, you might want also want to use Facebook's Prophet package (Auto.arima is easy to use, but two years of weekly data is bordering not enough data for an ARIMA model in my experience). Personally I have found Prophet to be easier to user when you have promotions and holiday event data available, otherwise ETS() might work better. Your real challenge is more of a coding challenge of how to efficiently iterate it over a large number of time series. You can check this response for more details on how to automate forecast generation.
In demand forecasting, some form of hierarchical forecasting is frequently performed. I.e you have 2000 products and you need a separate forecast for each separate product, but there are similarities between products that might help with the forecasting. You want to find some way of grouping the product together along a product hierarchy and then use hierarchical forecasting to improve accuracy. Since you are looking for forecasts at the individual product level, look at trying the top-down hierarchical approach.
Something a little bit more farfetched, but I would like call it out: Amazon and Uber use neural networks for this type of problem, where instead of having a separate forecast for each product/time series, they use one gigantic recurrent neural network to forecast all the time series in bulk. Note that they still end up with individual forecasts for each product (in Uber's case it is traffic/demand per city as opposed to products), they are just using a large model (an LSTM deep learning model) to do it all at once. The idea is similar in spirit to hierarchical forecasting in the sense that the neural network learns from the similarities between the histories of different products to come up with better forecasts. The Uber team has made some of their code available (through the M4 competition Github repositories), however it is C++ code (not exactly the favorite language of the stats crowd). Amazon's approach is not open source and you have to use their paid Amazon Forecast service to do the forecasts.
$endgroup$
A follow up to @StephanKolassa 's answer:
I concur with Prof Kolassa that ETS() from the forecast package in R is probably your best and fastest choice. If ETS doesn't give good results, you might want also want to use Facebook's Prophet package (Auto.arima is easy to use, but two years of weekly data is bordering not enough data for an ARIMA model in my experience). Personally I have found Prophet to be easier to user when you have promotions and holiday event data available, otherwise ETS() might work better. Your real challenge is more of a coding challenge of how to efficiently iterate it over a large number of time series. You can check this response for more details on how to automate forecast generation.
In demand forecasting, some form of hierarchical forecasting is frequently performed. I.e you have 2000 products and you need a separate forecast for each separate product, but there are similarities between products that might help with the forecasting. You want to find some way of grouping the product together along a product hierarchy and then use hierarchical forecasting to improve accuracy. Since you are looking for forecasts at the individual product level, look at trying the top-down hierarchical approach.
Something a little bit more farfetched, but I would like call it out: Amazon and Uber use neural networks for this type of problem, where instead of having a separate forecast for each product/time series, they use one gigantic recurrent neural network to forecast all the time series in bulk. Note that they still end up with individual forecasts for each product (in Uber's case it is traffic/demand per city as opposed to products), they are just using a large model (an LSTM deep learning model) to do it all at once. The idea is similar in spirit to hierarchical forecasting in the sense that the neural network learns from the similarities between the histories of different products to come up with better forecasts. The Uber team has made some of their code available (through the M4 competition Github repositories), however it is C++ code (not exactly the favorite language of the stats crowd). Amazon's approach is not open source and you have to use their paid Amazon Forecast service to do the forecasts.
answered 16 mins ago


Skander H.Skander H.
3,5631128
3,5631128
add a comment |
add a comment |
$begingroup$
Segmenting based on the variance of the original series makes no sense to me as the best model should be invariant to scale. Consider a series ..model it and then multiply each value in the time series by 1000 .
In terms of mass producing equations that may have both deterministic structure (pulses/level shift/local time trends ) OR either auto-regressive seasonality and arima structure you have to run a computer-based script . Beware of simple auto arima solutions that assume no deterministic structure OR fixed assumptions about same.
$endgroup$
add a comment |
$begingroup$
Segmenting based on the variance of the original series makes no sense to me as the best model should be invariant to scale. Consider a series ..model it and then multiply each value in the time series by 1000 .
In terms of mass producing equations that may have both deterministic structure (pulses/level shift/local time trends ) OR either auto-regressive seasonality and arima structure you have to run a computer-based script . Beware of simple auto arima solutions that assume no deterministic structure OR fixed assumptions about same.
$endgroup$
add a comment |
$begingroup$
Segmenting based on the variance of the original series makes no sense to me as the best model should be invariant to scale. Consider a series ..model it and then multiply each value in the time series by 1000 .
In terms of mass producing equations that may have both deterministic structure (pulses/level shift/local time trends ) OR either auto-regressive seasonality and arima structure you have to run a computer-based script . Beware of simple auto arima solutions that assume no deterministic structure OR fixed assumptions about same.
$endgroup$
Segmenting based on the variance of the original series makes no sense to me as the best model should be invariant to scale. Consider a series ..model it and then multiply each value in the time series by 1000 .
In terms of mass producing equations that may have both deterministic structure (pulses/level shift/local time trends ) OR either auto-regressive seasonality and arima structure you have to run a computer-based script . Beware of simple auto arima solutions that assume no deterministic structure OR fixed assumptions about same.
answered 5 hours ago


IrishStatIrishStat
20.7k42141
20.7k42141
add a comment |
add a comment |
Thanks for contributing an answer to Cross Validated!
- Please be sure to answer the question. Provide details and share your research!
But avoid …
- Asking for help, clarification, or responding to other answers.
- Making statements based on opinion; back them up with references or personal experience.
Use MathJax to format equations. MathJax reference.
To learn more, see our tips on writing great answers.
Sign up or log in
StackExchange.ready(function () {
StackExchange.helpers.onClickDraftSave('#login-link');
});
Sign up using Google
Sign up using Facebook
Sign up using Email and Password
Post as a guest
Required, but never shown
StackExchange.ready(
function () {
StackExchange.openid.initPostLogin('.new-post-login', 'https%3a%2f%2fstats.stackexchange.com%2fquestions%2f389291%2fstrategies-for-time-series-forecasting-for-2000-different-products%23new-answer', 'question_page');
}
);
Post as a guest
Required, but never shown
Sign up or log in
StackExchange.ready(function () {
StackExchange.helpers.onClickDraftSave('#login-link');
});
Sign up using Google
Sign up using Facebook
Sign up using Email and Password
Post as a guest
Required, but never shown
Sign up or log in
StackExchange.ready(function () {
StackExchange.helpers.onClickDraftSave('#login-link');
});
Sign up using Google
Sign up using Facebook
Sign up using Email and Password
Post as a guest
Required, but never shown
Sign up or log in
StackExchange.ready(function () {
StackExchange.helpers.onClickDraftSave('#login-link');
});
Sign up using Google
Sign up using Facebook
Sign up using Email and Password
Sign up using Google
Sign up using Facebook
Sign up using Email and Password
Post as a guest
Required, but never shown
Required, but never shown
Required, but never shown
Required, but never shown
Required, but never shown
Required, but never shown
Required, but never shown
Required, but never shown
Required, but never shown
9,ldgdIg6ESCol uU
$begingroup$
Are those similar products? You might benefit from searching this sitr for hierarchical forecasting
$endgroup$
– kjetil b halvorsen
4 hours ago