Structures for incorporating linear functions into a nonlinear optimization problem
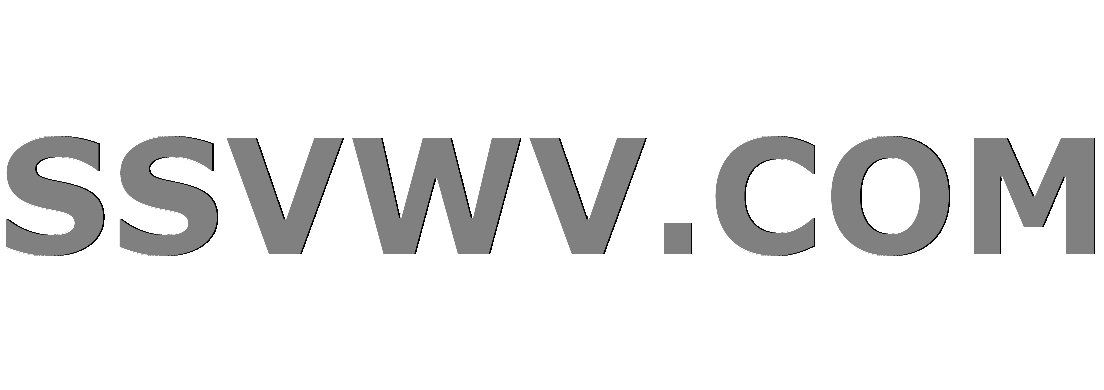
Multi tool use
$begingroup$
I'm working on a problem which naturally involves both linear and nonlinear operations, and I'd like some help understanding the best way to combine these into a neural network framework. To be more precise: suppose I have a matrix $A$, and some basis of matrices which it can be decomposed into, i.e.,
$$A = sum_{j}a_{j}A_{j}$$
for some real numbers $a_{j}$ and some pre-specified basis matrices $A_{j}$. Once I have this matrix $A$, I want to apply a function $f$ to it to obtain $f(A) = X$. Note that in this context I mean actually applying $f$ to the full matrix $A$: it is not elementwise applications of $f$, i.e., $f(A)$ is defined by the Taylor series of $f$, and $f$ is a nonlinear function. (In case it matters, the function I have in mind is the matrix exponential, but I don't think this should make much of a difference in the statement of the problem.)
Now, I also have some objective matrix $Y$. My goal is to find $A$ such that $X$ approximates $Y$ as closely as possible. The key here is that $f$ is a nonlinear function, but I want to restrict $A$ to be a linear combination of the $A_{j}$'s. I don't know how to incorporate that constraint naturally in a machine learning context, but it's obviously desirable to do so since I would like to do gradient descent over both the $a_{j}$'s and the parameters which enforce the function $f$ to find the desired matrix $X$. Any guidance, broadly speaking about what neural network architectures are best to tackle this kind of problem would be very helpful.
prediction linear-algebra
New contributor
mflynn is a new contributor to this site. Take care in asking for clarification, commenting, and answering.
Check out our Code of Conduct.
$endgroup$
add a comment |
$begingroup$
I'm working on a problem which naturally involves both linear and nonlinear operations, and I'd like some help understanding the best way to combine these into a neural network framework. To be more precise: suppose I have a matrix $A$, and some basis of matrices which it can be decomposed into, i.e.,
$$A = sum_{j}a_{j}A_{j}$$
for some real numbers $a_{j}$ and some pre-specified basis matrices $A_{j}$. Once I have this matrix $A$, I want to apply a function $f$ to it to obtain $f(A) = X$. Note that in this context I mean actually applying $f$ to the full matrix $A$: it is not elementwise applications of $f$, i.e., $f(A)$ is defined by the Taylor series of $f$, and $f$ is a nonlinear function. (In case it matters, the function I have in mind is the matrix exponential, but I don't think this should make much of a difference in the statement of the problem.)
Now, I also have some objective matrix $Y$. My goal is to find $A$ such that $X$ approximates $Y$ as closely as possible. The key here is that $f$ is a nonlinear function, but I want to restrict $A$ to be a linear combination of the $A_{j}$'s. I don't know how to incorporate that constraint naturally in a machine learning context, but it's obviously desirable to do so since I would like to do gradient descent over both the $a_{j}$'s and the parameters which enforce the function $f$ to find the desired matrix $X$. Any guidance, broadly speaking about what neural network architectures are best to tackle this kind of problem would be very helpful.
prediction linear-algebra
New contributor
mflynn is a new contributor to this site. Take care in asking for clarification, commenting, and answering.
Check out our Code of Conduct.
$endgroup$
add a comment |
$begingroup$
I'm working on a problem which naturally involves both linear and nonlinear operations, and I'd like some help understanding the best way to combine these into a neural network framework. To be more precise: suppose I have a matrix $A$, and some basis of matrices which it can be decomposed into, i.e.,
$$A = sum_{j}a_{j}A_{j}$$
for some real numbers $a_{j}$ and some pre-specified basis matrices $A_{j}$. Once I have this matrix $A$, I want to apply a function $f$ to it to obtain $f(A) = X$. Note that in this context I mean actually applying $f$ to the full matrix $A$: it is not elementwise applications of $f$, i.e., $f(A)$ is defined by the Taylor series of $f$, and $f$ is a nonlinear function. (In case it matters, the function I have in mind is the matrix exponential, but I don't think this should make much of a difference in the statement of the problem.)
Now, I also have some objective matrix $Y$. My goal is to find $A$ such that $X$ approximates $Y$ as closely as possible. The key here is that $f$ is a nonlinear function, but I want to restrict $A$ to be a linear combination of the $A_{j}$'s. I don't know how to incorporate that constraint naturally in a machine learning context, but it's obviously desirable to do so since I would like to do gradient descent over both the $a_{j}$'s and the parameters which enforce the function $f$ to find the desired matrix $X$. Any guidance, broadly speaking about what neural network architectures are best to tackle this kind of problem would be very helpful.
prediction linear-algebra
New contributor
mflynn is a new contributor to this site. Take care in asking for clarification, commenting, and answering.
Check out our Code of Conduct.
$endgroup$
I'm working on a problem which naturally involves both linear and nonlinear operations, and I'd like some help understanding the best way to combine these into a neural network framework. To be more precise: suppose I have a matrix $A$, and some basis of matrices which it can be decomposed into, i.e.,
$$A = sum_{j}a_{j}A_{j}$$
for some real numbers $a_{j}$ and some pre-specified basis matrices $A_{j}$. Once I have this matrix $A$, I want to apply a function $f$ to it to obtain $f(A) = X$. Note that in this context I mean actually applying $f$ to the full matrix $A$: it is not elementwise applications of $f$, i.e., $f(A)$ is defined by the Taylor series of $f$, and $f$ is a nonlinear function. (In case it matters, the function I have in mind is the matrix exponential, but I don't think this should make much of a difference in the statement of the problem.)
Now, I also have some objective matrix $Y$. My goal is to find $A$ such that $X$ approximates $Y$ as closely as possible. The key here is that $f$ is a nonlinear function, but I want to restrict $A$ to be a linear combination of the $A_{j}$'s. I don't know how to incorporate that constraint naturally in a machine learning context, but it's obviously desirable to do so since I would like to do gradient descent over both the $a_{j}$'s and the parameters which enforce the function $f$ to find the desired matrix $X$. Any guidance, broadly speaking about what neural network architectures are best to tackle this kind of problem would be very helpful.
prediction linear-algebra
prediction linear-algebra
New contributor
mflynn is a new contributor to this site. Take care in asking for clarification, commenting, and answering.
Check out our Code of Conduct.
New contributor
mflynn is a new contributor to this site. Take care in asking for clarification, commenting, and answering.
Check out our Code of Conduct.
New contributor
mflynn is a new contributor to this site. Take care in asking for clarification, commenting, and answering.
Check out our Code of Conduct.
asked 3 mins ago
mflynnmflynn
1
1
New contributor
mflynn is a new contributor to this site. Take care in asking for clarification, commenting, and answering.
Check out our Code of Conduct.
New contributor
mflynn is a new contributor to this site. Take care in asking for clarification, commenting, and answering.
Check out our Code of Conduct.
mflynn is a new contributor to this site. Take care in asking for clarification, commenting, and answering.
Check out our Code of Conduct.
add a comment |
add a comment |
0
active
oldest
votes
Your Answer
StackExchange.ifUsing("editor", function () {
return StackExchange.using("mathjaxEditing", function () {
StackExchange.MarkdownEditor.creationCallbacks.add(function (editor, postfix) {
StackExchange.mathjaxEditing.prepareWmdForMathJax(editor, postfix, [["$", "$"], ["\\(","\\)"]]);
});
});
}, "mathjax-editing");
StackExchange.ready(function() {
var channelOptions = {
tags: "".split(" "),
id: "557"
};
initTagRenderer("".split(" "), "".split(" "), channelOptions);
StackExchange.using("externalEditor", function() {
// Have to fire editor after snippets, if snippets enabled
if (StackExchange.settings.snippets.snippetsEnabled) {
StackExchange.using("snippets", function() {
createEditor();
});
}
else {
createEditor();
}
});
function createEditor() {
StackExchange.prepareEditor({
heartbeatType: 'answer',
autoActivateHeartbeat: false,
convertImagesToLinks: false,
noModals: true,
showLowRepImageUploadWarning: true,
reputationToPostImages: null,
bindNavPrevention: true,
postfix: "",
imageUploader: {
brandingHtml: "Powered by u003ca class="icon-imgur-white" href="https://imgur.com/"u003eu003c/au003e",
contentPolicyHtml: "User contributions licensed under u003ca href="https://creativecommons.org/licenses/by-sa/3.0/"u003ecc by-sa 3.0 with attribution requiredu003c/au003e u003ca href="https://stackoverflow.com/legal/content-policy"u003e(content policy)u003c/au003e",
allowUrls: true
},
onDemand: true,
discardSelector: ".discard-answer"
,immediatelyShowMarkdownHelp:true
});
}
});
mflynn is a new contributor. Be nice, and check out our Code of Conduct.
Sign up or log in
StackExchange.ready(function () {
StackExchange.helpers.onClickDraftSave('#login-link');
});
Sign up using Google
Sign up using Facebook
Sign up using Email and Password
Post as a guest
Required, but never shown
StackExchange.ready(
function () {
StackExchange.openid.initPostLogin('.new-post-login', 'https%3a%2f%2fdatascience.stackexchange.com%2fquestions%2f44890%2fstructures-for-incorporating-linear-functions-into-a-nonlinear-optimization-prob%23new-answer', 'question_page');
}
);
Post as a guest
Required, but never shown
0
active
oldest
votes
0
active
oldest
votes
active
oldest
votes
active
oldest
votes
mflynn is a new contributor. Be nice, and check out our Code of Conduct.
mflynn is a new contributor. Be nice, and check out our Code of Conduct.
mflynn is a new contributor. Be nice, and check out our Code of Conduct.
mflynn is a new contributor. Be nice, and check out our Code of Conduct.
Thanks for contributing an answer to Data Science Stack Exchange!
- Please be sure to answer the question. Provide details and share your research!
But avoid …
- Asking for help, clarification, or responding to other answers.
- Making statements based on opinion; back them up with references or personal experience.
Use MathJax to format equations. MathJax reference.
To learn more, see our tips on writing great answers.
Sign up or log in
StackExchange.ready(function () {
StackExchange.helpers.onClickDraftSave('#login-link');
});
Sign up using Google
Sign up using Facebook
Sign up using Email and Password
Post as a guest
Required, but never shown
StackExchange.ready(
function () {
StackExchange.openid.initPostLogin('.new-post-login', 'https%3a%2f%2fdatascience.stackexchange.com%2fquestions%2f44890%2fstructures-for-incorporating-linear-functions-into-a-nonlinear-optimization-prob%23new-answer', 'question_page');
}
);
Post as a guest
Required, but never shown
Sign up or log in
StackExchange.ready(function () {
StackExchange.helpers.onClickDraftSave('#login-link');
});
Sign up using Google
Sign up using Facebook
Sign up using Email and Password
Post as a guest
Required, but never shown
Sign up or log in
StackExchange.ready(function () {
StackExchange.helpers.onClickDraftSave('#login-link');
});
Sign up using Google
Sign up using Facebook
Sign up using Email and Password
Post as a guest
Required, but never shown
Sign up or log in
StackExchange.ready(function () {
StackExchange.helpers.onClickDraftSave('#login-link');
});
Sign up using Google
Sign up using Facebook
Sign up using Email and Password
Sign up using Google
Sign up using Facebook
Sign up using Email and Password
Post as a guest
Required, but never shown
Required, but never shown
Required, but never shown
Required, but never shown
Required, but never shown
Required, but never shown
Required, but never shown
Required, but never shown
Required, but never shown
LuoP7p1k,Wo4w,HiHVLdcM,21je6,0PWacEG kiDRpGLEI9D9wOcQ Nl3lCJ